Method for calculating molecular energy of ethane by deep learning
A deep learning and molecular technology, applied in chemical statistics, molecular entity identification, chemical machine learning, etc., can solve problems such as difficulty in obtaining satisfactory results, and achieve the effect of avoiding convergence problems and reducing impact.
- Summary
- Abstract
- Description
- Claims
- Application Information
AI Technical Summary
Problems solved by technology
Method used
Image
Examples
Embodiment 1
[0040] A method to calculate the energy of ethane molecules through deep learning, using the spatial coordinates and corresponding energies of 1000 different configurations of ethane molecules. In ethane molecule, the C-H bond length (r C1-H1 , r C1-H2 , r C1-H3 , r C2-H4 , r C2-H5 , r C2-H6 ) ranges from C-C bond length (r C1-C2 ) ranges from The bond angle formed by C-C-H in the molecule (θ C2-C1-H1 , θ C2-C1-H2 , θ C2-C1-H3 , θ C1-C2-H4 , θ C1-C2-H5 , θ C1-C2-H6 ,) The variation range is 111.2±5°; the bond angle formed by H-C-H (θ H1-C1-H2 , θ H1-C1-H3 , θ H2-C1-H3 , θ H4-C1-H5 , θ H4-C1-H6 , θ H5-C1-H6 ) range of 107.6±5°; each dihedral angle (φ H1-C1-C2-H4 , φ H1-C1-C2-H5 , φ H1-C1-C2-H6 , φ H2-C1-C2-H4 , φ H2-C1-C2-H5 , φ H2-C1-C2-H6 , φ H3-C1-C2-H4 , φ H3-C1-C2-H5 , φ H3-C1-C2-H6), the range of change is 0-360°. The molecular energies of ethane in different configurations were obtained by Quantum ESPRESSO chemical calculation software, and ...
Embodiment 2
[0070] This embodiment provides a preferred solution of the hardware platform and software environment of the present invention.
[0071] Choose the low-end i5-6500CPU@3.20GHz / NVIDIA Corporation GK208[GeForce GT730] / 4G Mem hardware platform to obtain higher general performance; the software environment is Linux kernel 4.9 / TensorFlow-GPU 1.8.0 (installed via pip ), the driver is CUDA 9.0 / cuDNN 7.1.
Embodiment 3
[0073] This embodiment provides a preferred scheme for selecting input data in the present invention.
[0074] The constructed dataset contains configurations of 1000 ethane molecules and their one-to-one corresponding energies. The configurations are expressed in Bohrpositions, and the energy unit is kJ / mol. This method avoids using the gradient descent method to calculate the minimized total energy while ensuring that the accuracy of calculating molecular energies increases as the training set increases, and compares the results with those calculated using the standard DFT approximation (PBE). The parameters of the ethane molecule are set to 11, which are: ∑r C-H , r C1-C2 , Σθ C-C-H , Σθ H-C-H ,∑cos3φ H-C-C-H , eleven parameters. Based on the PBE results, the optimized ethane molecular configuration (C-H bond length is The bond length of C-C bond is The bond angle formed by C-C-H is 111.2°; the bond angle formed by H-C-H is 107.6°; each dihedral angle i...
PUM
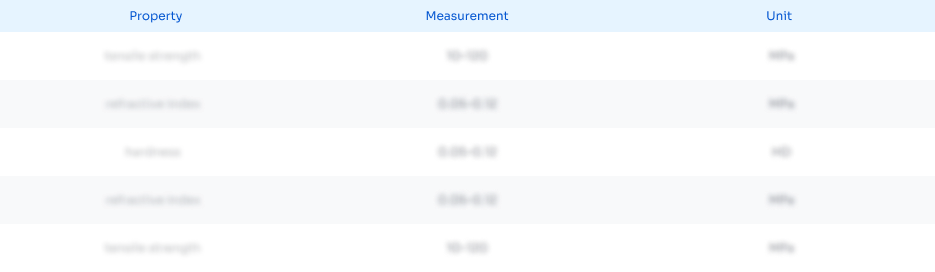
Abstract
Description
Claims
Application Information
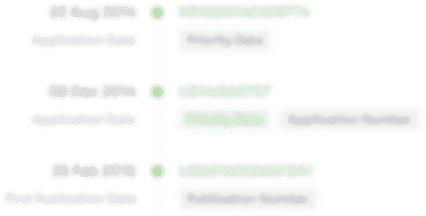
- R&D Engineer
- R&D Manager
- IP Professional
- Industry Leading Data Capabilities
- Powerful AI technology
- Patent DNA Extraction
Browse by: Latest US Patents, China's latest patents, Technical Efficacy Thesaurus, Application Domain, Technology Topic, Popular Technical Reports.
© 2024 PatSnap. All rights reserved.Legal|Privacy policy|Modern Slavery Act Transparency Statement|Sitemap|About US| Contact US: help@patsnap.com