A machine learning method for locally missing multi-view clustering based on matrix-guided regularization
A machine learning and multi-view technology, applied in the field of computer vision and pattern recognition, can solve problems such as ineffective use of kernel matrix, high kernel redundancy, and affecting clustering performance, so as to avoid unreliable similarity evaluation , Reduce high redundancy, good clustering effect
- Summary
- Abstract
- Description
- Claims
- Application Information
AI Technical Summary
Problems solved by technology
Method used
Image
Examples
Embodiment Construction
[0046] The present invention will be described in detail below in conjunction with the accompanying drawings and specific embodiments. This embodiment is carried out on the premise of the technical solution of the present invention, and detailed implementation and specific operation process are given, but the protection scope of the present invention is not limited to the following embodiments.
[0047] 1. Multi-core k-means algorithm (MKKM)
[0048] Represents a collection of n samples, Indicates that the pth feature is matched by x to a regenerated kernel Hilbert space In a multi-core configuration, each sample has multiple feature representations, which are represented by a set of feature maps Defined. Specifically, each sample is denoted as φ β (x)=[β 1 φ 1 (x) T ,...,β m φ m (x) T ] T , where β=[β 1 ,...,β m ] T , representing the coefficients of the m base kernels. These coefficients will be optimized during the learning process. Based on the definit...
PUM
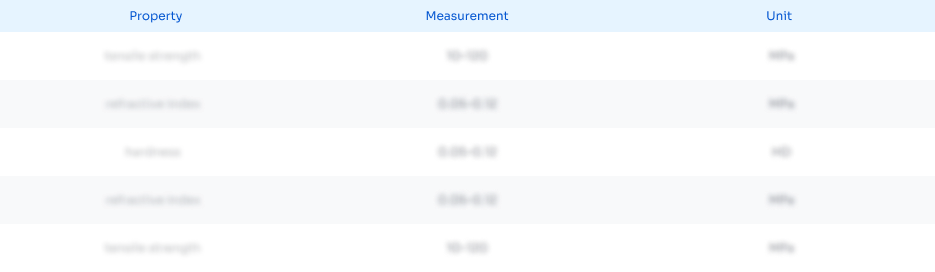
Abstract
Description
Claims
Application Information
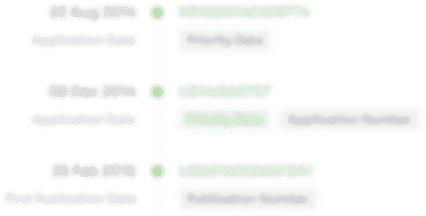
- R&D
- Intellectual Property
- Life Sciences
- Materials
- Tech Scout
- Unparalleled Data Quality
- Higher Quality Content
- 60% Fewer Hallucinations
Browse by: Latest US Patents, China's latest patents, Technical Efficacy Thesaurus, Application Domain, Technology Topic, Popular Technical Reports.
© 2025 PatSnap. All rights reserved.Legal|Privacy policy|Modern Slavery Act Transparency Statement|Sitemap|About US| Contact US: help@patsnap.com