A lightweight color depth learning model for near-infrared images with fusion layer
A near-infrared and colorization technology, applied in image data processing, 2D image generation, biological neural network models, etc., can solve the problems that scene images cannot be colored, equipment hardware requirements are extremely high, and training time increases, etc., to achieve The effect of rich image details, simple and practical method, and consistent object color
- Summary
- Abstract
- Description
- Claims
- Application Information
AI Technical Summary
Problems solved by technology
Method used
Image
Examples
Embodiment Construction
[0021] The process of this method is as follows figure 1 As shown, the method first uses the lightweight Faseter-RCNN image recognition module to recognize near-infrared images, and then searches for 500 images similar to the scene in the entire network, uses this as a training set, and enters the colorized network The module is trained, and finally the near-infrared image is input as the test set to complete the image colorization. The network structure, process and steps of the technical solution of the method will be described below in conjunction with the accompanying drawings.
[0022] 1. Image recognition network module
[0023] The image recognition network model structure is as figure 2 Shown. ①First input the NIR image to VGG-16; ②NIR is propagated forward through CNN to the last shared convolutional layer, and the feature map for RPN network input is obtained, and then forward propagated to the last convolutional layer to generate higher-dimensional feature maps ③The fe...
PUM
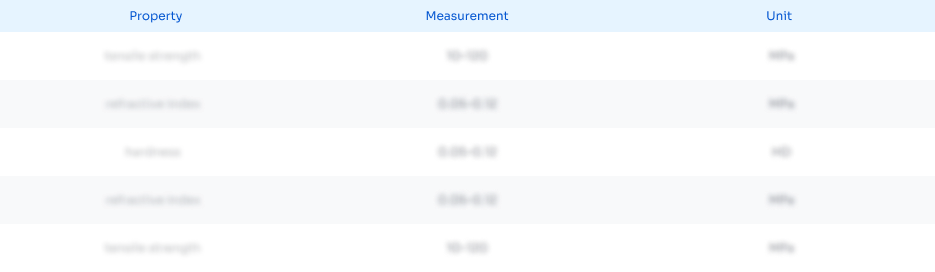
Abstract
Description
Claims
Application Information
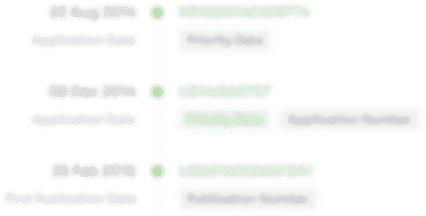
- R&D Engineer
- R&D Manager
- IP Professional
- Industry Leading Data Capabilities
- Powerful AI technology
- Patent DNA Extraction
Browse by: Latest US Patents, China's latest patents, Technical Efficacy Thesaurus, Application Domain, Technology Topic, Popular Technical Reports.
© 2024 PatSnap. All rights reserved.Legal|Privacy policy|Modern Slavery Act Transparency Statement|Sitemap|About US| Contact US: help@patsnap.com