A method and system for counteracting cross-modal retrieval based on dictionary learning
A dictionary learning and cross-modal technology, applied in the field of cross-modal retrieval, can solve the problems of not having the maximum correlation, ignoring the statistical characteristics of multi-modal data, and unable to maintain the inherent statistical characteristics of the original characteristics of the modality
- Summary
- Abstract
- Description
- Claims
- Application Information
AI Technical Summary
Problems solved by technology
Method used
Image
Examples
Embodiment 1
[0059] This embodiment provides an adversarial cross-modal retrieval method based on dictionary learning, and the specific steps are as follows:
[0060] Step S1: Obtain the underlying features of images and texts, construct a data set including image modalities and text modalities and their semantic labels, and divide them into image training set, text training set, image test set and text test set.
[0061] The image training set is denoted as d v is the image feature dimension, and m is the number of samples. The text training set is denoted as d t is the text feature dimension, and m is the number of samples. X, Y are feature matrices. The image-text pairs in the training set are denoted as P={X,Y}. In the same way, we can divide the test set X of images and text te , Y te .
[0062] Taking the Wikipedia-CNN dataset as an example, the Wikipedia-CNN dataset contains 2866 image-text pairs and their corresponding semantic labels. 2173 image-text pairs are randomly...
Embodiment 2
[0104] The purpose of this embodiment is to provide a computing system.
[0105] A confrontational cross-modal retrieval system based on dictionary learning, comprising a memory, a processor, and a computer program stored on the memory and operable on the processor, and the processor implements the following steps when executing the program, including:
[0106] Obtaining the underlying features of image data and text data, and constructing a training set and a test set of images and text respectively based on the underlying features;
[0107] Construct a dictionary learning model, train based on image and text training sets, and obtain image dictionaries, text dictionaries, image reconstruction coefficients and text reconstruction coefficients;
[0108] According to the image dictionary and the text dictionary, calculate the image reconstruction coefficient and the text reconstruction coefficient of the test set;
[0109] The image reconstruction coefficient and text reconstr...
Embodiment 3
[0114] The purpose of this embodiment is to provide a computer-readable storage medium.
[0115] A computer-readable storage medium, on which a computer program is stored, and when the program is executed by a processor, the following steps are performed:
[0116] Obtaining the underlying features of image data and text data, and constructing a training set and a test set of images and text respectively based on the underlying features;
[0117] Construct a dictionary learning model, train based on image and text training sets, and obtain image dictionaries, text dictionaries, image reconstruction coefficients and text reconstruction coefficients;
[0118] According to the image dictionary and the text dictionary, calculate the image reconstruction coefficient and the text reconstruction coefficient of the test set;
[0119] The image reconstruction coefficient and text reconstruction coefficient of the training set, and the transposed form of the image reconstruction coeffic...
PUM
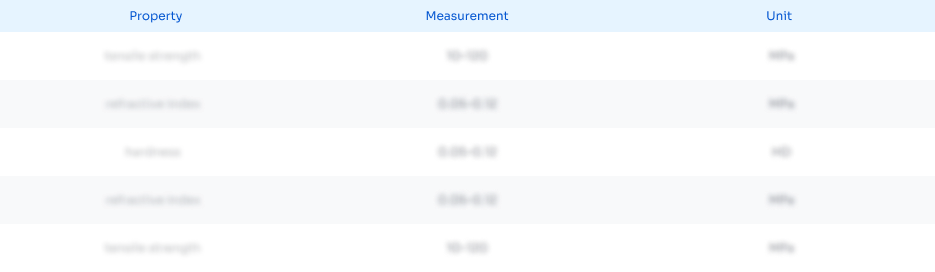
Abstract
Description
Claims
Application Information
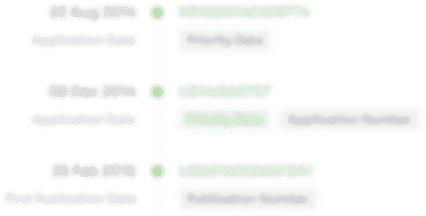
- R&D Engineer
- R&D Manager
- IP Professional
- Industry Leading Data Capabilities
- Powerful AI technology
- Patent DNA Extraction
Browse by: Latest US Patents, China's latest patents, Technical Efficacy Thesaurus, Application Domain, Technology Topic, Popular Technical Reports.
© 2024 PatSnap. All rights reserved.Legal|Privacy policy|Modern Slavery Act Transparency Statement|Sitemap|About US| Contact US: help@patsnap.com