A method of building remote sensing image recognition based on convolution neural network
A convolutional neural network and remote sensing image technology, which is applied in the field of remote sensing image supervision and classification, can solve the problems of not achieving automation, affecting the accuracy of building outlines, and the accuracy of segmentation results is not very good.
- Summary
- Abstract
- Description
- Claims
- Application Information
AI Technical Summary
Problems solved by technology
Method used
Image
Examples
Embodiment
[0054] A method for building remote sensing image recognition based on convolutional neural network, such as figure 1 shown, including the following steps:
[0055] Step 1: Obtain the original remote sensing image; the original remote sensing image is downloaded from the remote sensing image data website or captured by the drone, choose one of the two;
[0056] The second step: radiometrically calibrate the original remote sensing image to obtain the radiometrically calibrated remote sensing image; the radiometric calibration, the specific process is: use the radiometric calibration tool to obtain the parameters in the original remote sensing image setting panel, and complete the radiometric calibration mark;
[0057] Step 3: Perform atmospheric correction on the radiometrically calibrated remote sensing image to obtain the atmospherically corrected remote sensing image; label each pixel of the atmospherically corrected remote sensing image to obtain the corresponding label ...
PUM
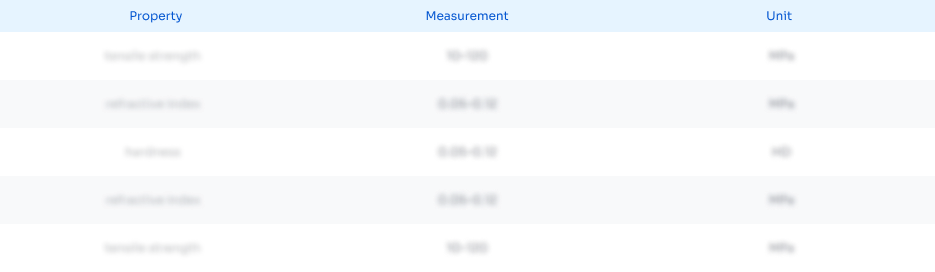
Abstract
Description
Claims
Application Information
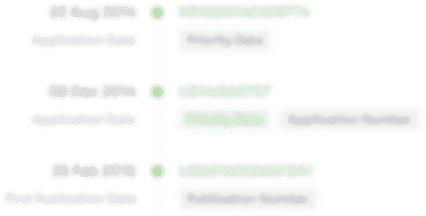
- R&D Engineer
- R&D Manager
- IP Professional
- Industry Leading Data Capabilities
- Powerful AI technology
- Patent DNA Extraction
Browse by: Latest US Patents, China's latest patents, Technical Efficacy Thesaurus, Application Domain, Technology Topic.
© 2024 PatSnap. All rights reserved.Legal|Privacy policy|Modern Slavery Act Transparency Statement|Sitemap