A deep learning high under-sampling hyperpolarized gas lung MRI reconstruction method
A deep learning and undersampling technology, applied in neural learning methods, 2D image generation, image data processing, etc., can solve problems such as difficulty in accurately estimating noise
- Summary
- Abstract
- Description
- Claims
- Application Information
AI Technical Summary
Problems solved by technology
Method used
Image
Examples
Embodiment 1
[0050] A high undersampling hyperpolarized gas lung MRI reconstruction method based on deep learning, comprising the following steps:
[0051] Step 1, constructing hyperpolarized gas lung MRI image training set, hyperpolarized gas in this embodiment is 129 Xe, the training set of hyperpolarized gas lung MRI images is 129 Xe lung MRI image training set;
[0052] In step 1.1, fully sampled hyperpolarized gas lung magnetic resonance k-space data and corresponding proton images were collected from 72 volunteers. The full-sampled hyperpolarized gas lung magnetic resonance k-space data were acquired by 3D bSSFP sequence, the sampling matrix size was 96×84, and the number of slices was 24. Fast Fourier transform is performed on the full-sampled hyperpolarized gas lung magnetic resonance k-space data, and the obtained reconstruction result is used as the reference image y, and the images whose signal area of the lung is smaller than 10% of the image size are eliminated, and a tota...
PUM
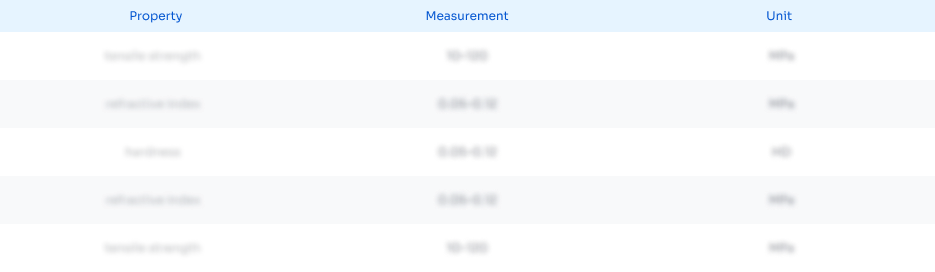
Abstract
Description
Claims
Application Information
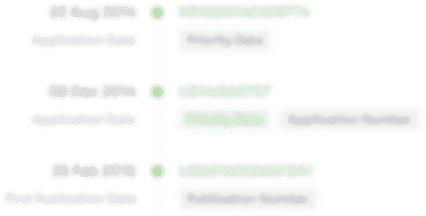
- R&D Engineer
- R&D Manager
- IP Professional
- Industry Leading Data Capabilities
- Powerful AI technology
- Patent DNA Extraction
Browse by: Latest US Patents, China's latest patents, Technical Efficacy Thesaurus, Application Domain, Technology Topic, Popular Technical Reports.
© 2024 PatSnap. All rights reserved.Legal|Privacy policy|Modern Slavery Act Transparency Statement|Sitemap|About US| Contact US: help@patsnap.com