Virtual water quality monitoring method based on improved depth limit learning machine
An extreme learning machine and water quality monitoring technology, which is applied in the direction of testing water and material inspection products, can solve the problems of insufficient ability to propose high-level features of water quality spatial data, and achieve the effect of improving generalization ability and prediction accuracy
- Summary
- Abstract
- Description
- Claims
- Application Information
AI Technical Summary
Problems solved by technology
Method used
Image
Examples
Embodiment Construction
[0028] The specific implementation process of the present invention is as follows:
[0029] The present invention mainly includes two parts: ELM-CDAE and a deep extreme learning machine based on ELM-CDAE.
[0030] Such as figure 1 As shown, the present invention introduces the local noise reduction criterion and the shrinking regular term into the autoencoder based on the extreme learning machine to form the ELM-CDAE. The training process of a single ELM-CDAE can be detailed as follows:
[0031] Step 1: Initialize the ELM-CDAE model: the selected interference noise is Gaussian noise, the number of hidden layer nodes is 8, and the input weights and biases are randomly generated.
[0032] Step 2: Use noise to add noise to the input water quality data x to obtain the noise input And make The statistical distribution satisfies
[0033] Step 3: Calculate the hidden layer output H:
[0034]
[0035] Where N is the number of samples, l is the number of hidden layer nodes, a j ,b j ,x i The...
PUM
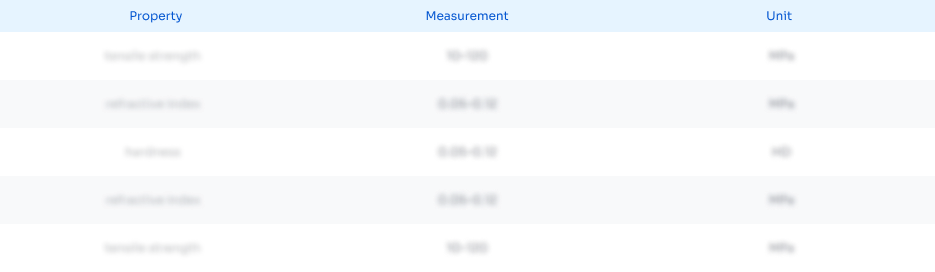
Abstract
Description
Claims
Application Information
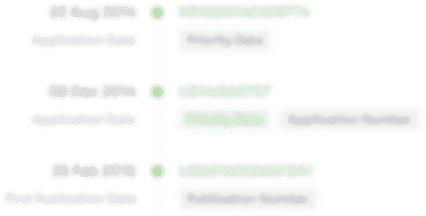
- R&D
- Intellectual Property
- Life Sciences
- Materials
- Tech Scout
- Unparalleled Data Quality
- Higher Quality Content
- 60% Fewer Hallucinations
Browse by: Latest US Patents, China's latest patents, Technical Efficacy Thesaurus, Application Domain, Technology Topic, Popular Technical Reports.
© 2025 PatSnap. All rights reserved.Legal|Privacy policy|Modern Slavery Act Transparency Statement|Sitemap|About US| Contact US: help@patsnap.com