A method and system for classifying and locating pulmonary nodules based on multi-slice CT images
A technology of CT image and positioning method, which is applied in the fields of computer vision and biomedicine, can solve the problem of difficult positioning of class activation value mapping, and achieve the effect of improving accuracy
- Summary
- Abstract
- Description
- Claims
- Application Information
AI Technical Summary
Problems solved by technology
Method used
Image
Examples
experiment example 1
[0077] Experiment 1: Model prediction performance and mitigation of overfitting
[0078]This part of the experiment used a variety of deep learning models, and conducted experiments on all the data sets in Table 1. It can be seen from Table 2 that, in terms of accuracy, with the increase of the number of sample channels, the accuracy rate has been significantly improved; but the result of 21-channel data is slightly lower than that of 11-channel, one possible reason is that with the increase of the number of channels The increase of , the two types of samples will contain more "background" information, which makes the features extracted by the model confuse the classifier, resulting in a decline in performance. Depend on Figure 6 ~ Figure 9 It can be seen that as the number of channels increases, the overfitting phenomenon has been significantly alleviated. The model of the present invention improves the accuracy rate by maintaining fine-grained lesion points, and obtains a...
experiment example 2
[0079] Experimental example 2: Comparison of soft activation mapping of different models
[0080] use image 3 In this part of the experiment, the relevant network model was modified, so that the final convolutional layer obtained a larger-sized feature map (16×16), and through soft activation mapping, a finer-grained focus point location was obtained. ( Figure 4 ). Compared with class activation mapping, "fine-grained" is more obvious in malignant lesions, but it will also lead to some false positives and false negatives.
experiment example 3
[0081] Experimental Example 3: Soft activation mapping for high-level feature enhancement
[0082] In this part of the experiment, the high-level and low-dimensional semantic features of the U-Net structure in the model of the present invention are added element-by-element to the vector obtained by soft activation mapping through global maximum pooling, and the category information is further integrated into the lesion location. Therefore, the soft activation mapping enhanced by high-level semantic features not only achieves finer-grained positioning, but also more accurate positioning, which improves the accuracy of classification. Depend on Figure 5 It can be seen that for typical solid nodules, hollow nodules, ground glass nodules and micro nodules and other typical lesions, the method of the present invention can locate more accurately, and greatly reduce false positive and false negative phenomena.
[0083] Table 1: Datasets containing samples with different number of c...
PUM
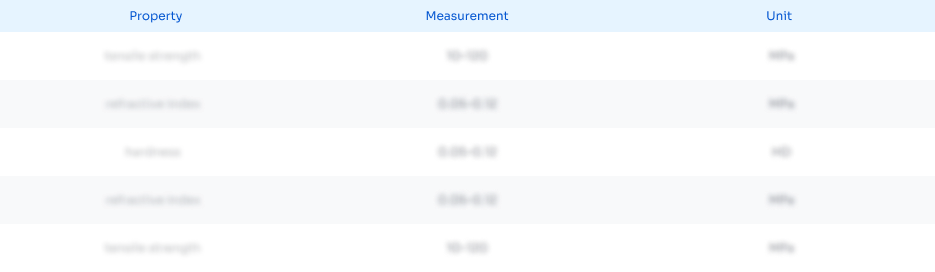
Abstract
Description
Claims
Application Information
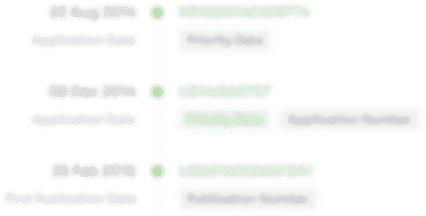
- R&D Engineer
- R&D Manager
- IP Professional
- Industry Leading Data Capabilities
- Powerful AI technology
- Patent DNA Extraction
Browse by: Latest US Patents, China's latest patents, Technical Efficacy Thesaurus, Application Domain, Technology Topic.
© 2024 PatSnap. All rights reserved.Legal|Privacy policy|Modern Slavery Act Transparency Statement|Sitemap