Parallel method of object detection and semantic segmentation based on end-to-end depth learning
A target detection and deep learning technology, applied in the field of computer vision of artificial intelligence, can solve the problems of lack of pertinence and manual design, division of target object boundary information, lack of diversity changes, etc., to achieve real-time target detection and target segmentation. Effect
- Summary
- Abstract
- Description
- Claims
- Application Information
AI Technical Summary
Problems solved by technology
Method used
Image
Examples
Embodiment 1
[0034] Such as Figure 1-2 As shown, a parallel method of object detection and semantic segmentation based on end-to-end deep learning includes the following steps:
[0035] Darknet-19 of S1 construction and training target detection branch
[0036] S1.1 Collecting images: Download the PASCAL VOC data set from the Internet, which provides a set of standard excellent data sets for image detection and image segmentation. This method uses this data set to fine-tune and test the model;
[0037]S1.2 Preprocessing the target data set: Use common scale transformation, random cropping, noise addition, and rotation transformation to preprocess the image to enhance the robustness of the model; this step makes the input scale of the target data set fixed from the beginning The size is randomly transformed to the size of n*32, and the value range of n is 9-19; the default input scale is 618;
[0038] S1.3 Generating feature images: The basic network structure used by Darknet-19 does not...
PUM
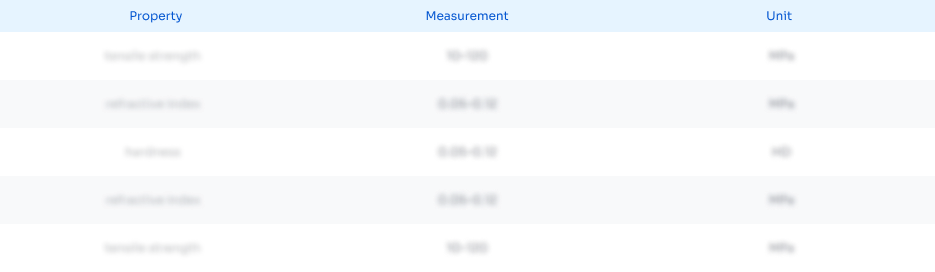
Abstract
Description
Claims
Application Information
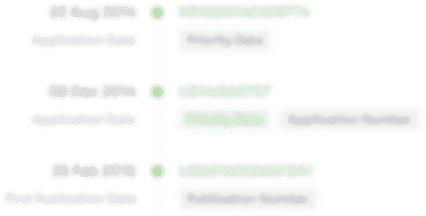
- R&D Engineer
- R&D Manager
- IP Professional
- Industry Leading Data Capabilities
- Powerful AI technology
- Patent DNA Extraction
Browse by: Latest US Patents, China's latest patents, Technical Efficacy Thesaurus, Application Domain, Technology Topic, Popular Technical Reports.
© 2024 PatSnap. All rights reserved.Legal|Privacy policy|Modern Slavery Act Transparency Statement|Sitemap|About US| Contact US: help@patsnap.com