A feature selection method for clustering algorithm based on density clustering
A feature selection method and clustering algorithm technology, applied in the field of data analysis, can solve the problems of high computational cost, overfitting, and low accuracy, and achieve the effects of accurate clustering results, improved processing capacity, and reduced computational cost.
- Summary
- Abstract
- Description
- Claims
- Application Information
AI Technical Summary
Problems solved by technology
Method used
Image
Examples
Embodiment 1
[0065] Example 1. A feature selection method for clustering algorithms based on density clustering, which is completed in the following steps,
[0066] a. Suppose: the data set D contains M instances and N features, then there is a feature set F={f consisting of N features 1 , f 2 ,..., f N};
[0067] Normalize the data set D to obtain the data set D', and then use the Euclidean distance (Euclidean distance) as the similarity measure between the features in the data set D' to construct a similarity matrix between the features; normalize Optimization can improve the accuracy rate, and it has a significant effect when it comes to the algorithm of distance calculation;
[0068] b. Use the DBSCAN algorithm to cluster the features of the similarity matrix, and divide the features into three categories: core features, boundary features, and atypical features; specifically, according to the principle of dividing points in the DBSCAN algorithm, the features are divided into three ...
PUM
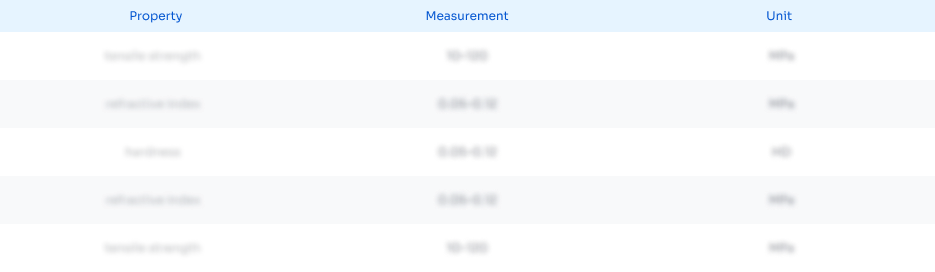
Abstract
Description
Claims
Application Information
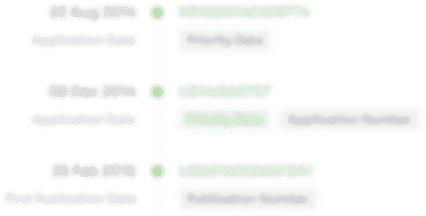
- Generate Ideas
- Intellectual Property
- Life Sciences
- Materials
- Tech Scout
- Unparalleled Data Quality
- Higher Quality Content
- 60% Fewer Hallucinations
Browse by: Latest US Patents, China's latest patents, Technical Efficacy Thesaurus, Application Domain, Technology Topic, Popular Technical Reports.
© 2025 PatSnap. All rights reserved.Legal|Privacy policy|Modern Slavery Act Transparency Statement|Sitemap|About US| Contact US: help@patsnap.com