An urban rail transit passenger flow volume prediction method based on A-LSTM
A technology of urban rail transit and forecasting method, which is applied in the field of urban rail transit passenger flow forecasting, can solve problems such as unobvious regularity, difficult prediction, and difficult prediction of rail transit passenger flow, and achieve the effect of improving prediction accuracy
- Summary
- Abstract
- Description
- Claims
- Application Information
AI Technical Summary
Problems solved by technology
Method used
Image
Examples
Embodiment 1
[0048] This embodiment mainly preprocesses the time distribution characteristics in step S3 of the present invention, adopts a hierarchical clustering method to cluster and analyze the time distribution characteristics, and further defines different sample types:
[0049] Firstly, hierarchical clustering is carried out on the time distribution characteristics. For a batch of samples studied in the present invention, there are multiple observation indicators, and there are different degrees of similarity between the indicators, so a cluster analysis method is proposed. This method aggregates samples (or indicators) with a large degree of similarity into one category, among which, the closely related samples (or indicators) are aggregated into a small taxonomic unit, and the distantly related samples are aggregated into a large taxonomic unit, thus forming different division types, and obtaining A classification system from small to large, and finally presents the relationship be...
Embodiment 2
[0058] This embodiment is mainly to further illustrate the long-short-term memory neural network A-LSTM model based on the attention mechanism of the present invention:
[0059] Bring the clustered historical traffic data with spatial and temporal features obtained in the previous step into the A-LSTM model for training. in, image 3 For the specific structure of LSTM, it is assumed that the input data of historical passenger flow data and spatio-temporal features are: x=(x 1 , x 2 ,...,x T ), the sequence of LSTM to calculate the hidden layer vector is: h=(h 1 , h 2 ,...,h T ), the real passenger flow data is y=(y 1 ,y 2 ,...,y T ), the predicted value is iteratively obtained by the following equation:
[0060] h t =H(W xh x t +W hh h t-1 +Z t-1 +b h ) (1)
[0061] Among them, W xh Represents the weight matrix of hidden layer input, W hh Represents the weight matrix of the hidden layer state input, b h is the hidden layer bias vector; H is the hidden layer...
Embodiment 3
[0069] On the basis of the first two embodiments, as an optional method, the attention model used in the present invention is as follows Figure 4 As shown, the model requires n hidden layer states: h=(h 1 , h 2 ,..., h n ) and passenger flow data x=(x1 ,x 2 ,...,x n ); return vector z, return vector z can be understood as attention mechanism value Z t collection.
[0070] The A-LSTM model constructed by the present invention is as Figure 5 As shown, a layer of attention mechanism is added to the LSTM network to receive the hidden layer state and the input passenger flow data, so as to dynamically adjust the weight of the input passenger flow data.
PUM
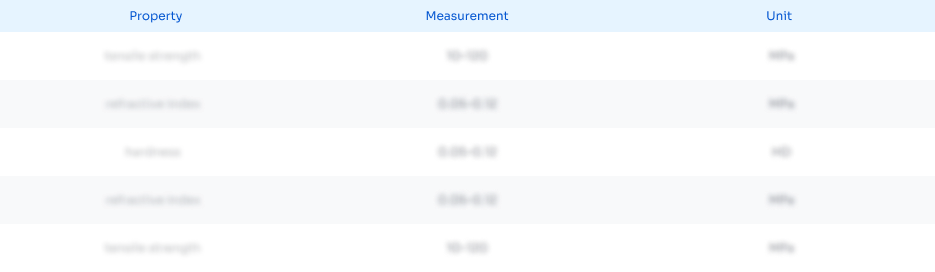
Abstract
Description
Claims
Application Information
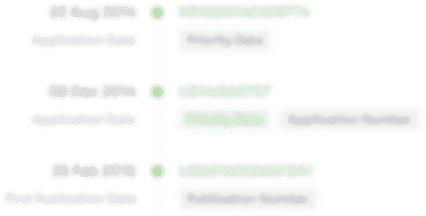
- R&D Engineer
- R&D Manager
- IP Professional
- Industry Leading Data Capabilities
- Powerful AI technology
- Patent DNA Extraction
Browse by: Latest US Patents, China's latest patents, Technical Efficacy Thesaurus, Application Domain, Technology Topic.
© 2024 PatSnap. All rights reserved.Legal|Privacy policy|Modern Slavery Act Transparency Statement|Sitemap