A short-term traffic prediction method based on multi-task multi-view learning model
A traffic forecasting and learning model technology, applied in the field of information technology services, can solve the problems of lack of rationality, difficulty in determining the model structure, ignoring the global correlation of geographical units, etc., and achieve the effect of reasonable method and balanced global forecasting ability
- Summary
- Abstract
- Description
- Claims
- Application Information
AI Technical Summary
Problems solved by technology
Method used
Image
Examples
Embodiment Construction
[0034] The present invention will be further described in detail below in conjunction with the accompanying drawings and specific embodiments.
[0035] Such as figure 1 As shown, the overall step flow chart of the present invention is shown, specifically:
[0036] Step 1, constructing a spatio-temporal data model for each road segment separately;
[0037]The spatio-temporal data model includes a spatial dimension and a temporal dimension, where the spatial dimension represents the number of spatial neighbors that affect the target road segment, and the temporal dimension represents the time window length that affects the historical traffic conditions at the current moment. Therefore, the key to determining the spatio-temporal data model lies in how to select the appropriate spatial neighbors and time window length. For the traffic conditions of each road segment at any time, the space-time proximity matrix, space-time period matrix, and space-time trend matrix can be used to...
PUM
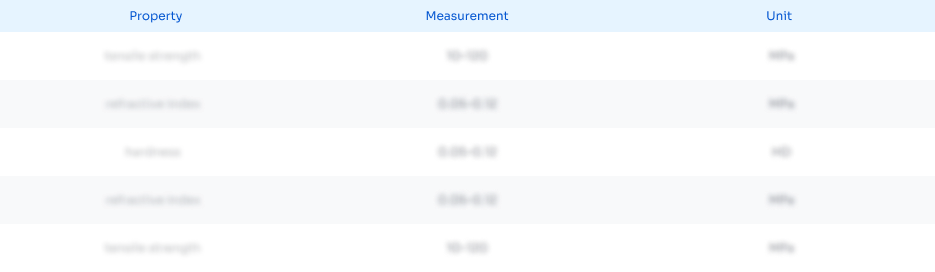
Abstract
Description
Claims
Application Information
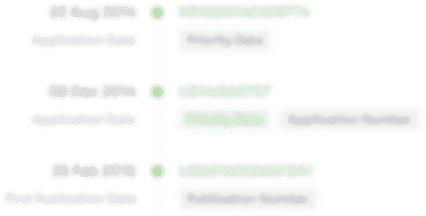
- R&D Engineer
- R&D Manager
- IP Professional
- Industry Leading Data Capabilities
- Powerful AI technology
- Patent DNA Extraction
Browse by: Latest US Patents, China's latest patents, Technical Efficacy Thesaurus, Application Domain, Technology Topic, Popular Technical Reports.
© 2024 PatSnap. All rights reserved.Legal|Privacy policy|Modern Slavery Act Transparency Statement|Sitemap|About US| Contact US: help@patsnap.com