Multi-modal emotion recognition method based on attention feature fusion
A feature fusion and emotion recognition technology, applied in the field of emotional computing, can solve the problems of not being able to reflect the degree of modal influence, ignoring the differences of different modal features, etc., to achieve maximum information utilization, simple and effective execution, and improve recognition effect Effect
- Summary
- Abstract
- Description
- Claims
- Application Information
AI Technical Summary
Problems solved by technology
Method used
Image
Examples
Embodiment 1
[0064] A method for multimodal emotion recognition based on attention feature fusion, comprising the following steps:
[0065] (1) Preprocessing the data of multiple modalities to make it meet the input requirements of the models corresponding to multiple modalities;
[0066] (2) feature extraction is carried out to the data of multiple modalities after step (1) preprocessing;
[0067] (3) Perform feature fusion of the data features of multiple modalities extracted in step (2): traditional feature layer fusion is to concatenate the feature vectors of the three modalities to form a total joint feature vector, and then Sent to the classifier for classification. However, since the characteristics of different modalities have different influences on our final recognition effect, in order to effectively obtain the influence weight of each modal feature on the final result according to the distribution of the data set. The attention mechanism is used to assign a weight to the data...
Embodiment 2
[0070] A kind of method based on the multimodal emotion recognition of attention feature fusion described in embodiment 1, such as figure 1 As shown, the difference is that in the step (1), the data of multiple modes includes text data, voice data, video data,
[0071] For text data, the preprocessing process includes: converting text data into mathematical data by training word vectors, that is, converting the words in each piece of text into a word vector representation, so that it meets the input requirements of the bidirectional LSTM model; bidirectional LSTM model It includes the word vector layer, the bidirectional LSTM layer, the first Dropout layer and the first fully connected layer in turn. The word vector layer is used to convert each word in the text into a word vector representation. The bidirectional LSTM layer is used to extract text features. The first Dropout The layer is used to avoid overfitting of the bidirectional LSTM model, and the first fully connected ...
PUM
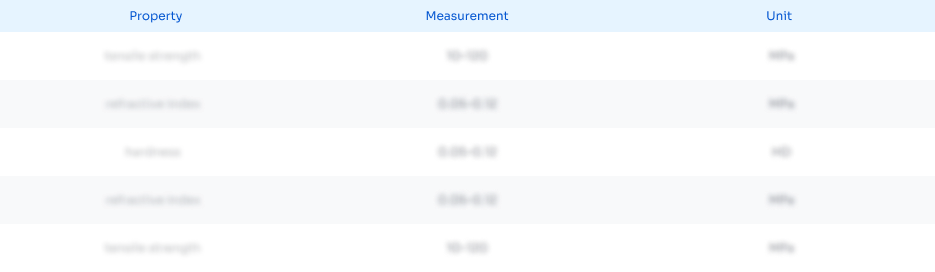
Abstract
Description
Claims
Application Information
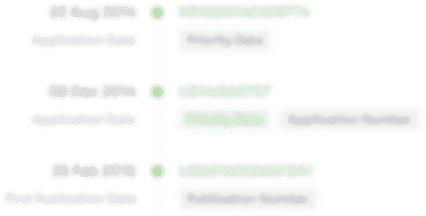
- R&D Engineer
- R&D Manager
- IP Professional
- Industry Leading Data Capabilities
- Powerful AI technology
- Patent DNA Extraction
Browse by: Latest US Patents, China's latest patents, Technical Efficacy Thesaurus, Application Domain, Technology Topic.
© 2024 PatSnap. All rights reserved.Legal|Privacy policy|Modern Slavery Act Transparency Statement|Sitemap