Rolling bearing fault intelligent diagnosis method based on improved S-transformation and deep learning
A technology for rolling bearings and fault diagnosis, which is applied in neural learning methods, testing of mechanical components, testing of machine/structural components, etc., can solve problems such as not being well applicable and not well adapting to bearing vibration signals, and achieve improvement The effect of accuracy
- Summary
- Abstract
- Description
- Claims
- Application Information
AI Technical Summary
Problems solved by technology
Method used
Image
Examples
Embodiment example 1
[0068] Take the bearing data of a university as an example to illustrate the implementation method of rolling bearing fault diagnosis based on the combination of improved S-transform and deep learning.
[0069] (1) Test data
[0070] Such as Figure 5 As shown, the rolling bearing experiment platform includes a 2 horsepower motor (left side) (1h=746w), a torque sensor (middle), a power meter (right side) and electronic control equipment. The test bench includes a drive end bearing and a fan end bearing, and the acceleration sensor is respectively installed at the 12 o'clock position of the drive end and the fan end of the motor housing. The vibration signal is collected by a 16-channel DAT recorder, and the sampling frequency of the drive end bearing fault data is 12kHz. In this experiment, the present invention selects the driving end (bearing) as the research object. When the motor load is 0, the bearing failure modes are selected as inner ring failure, outer ring failure and r...
PUM
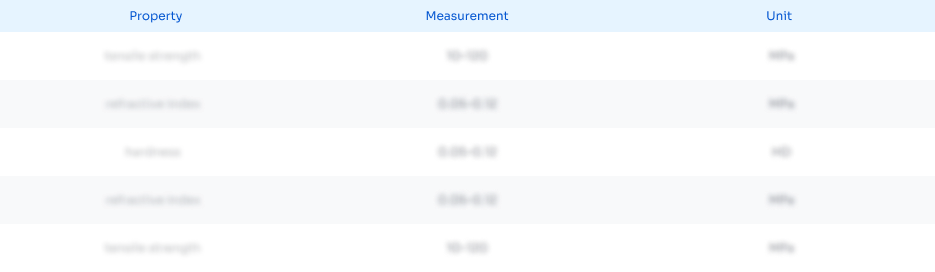
Abstract
Description
Claims
Application Information
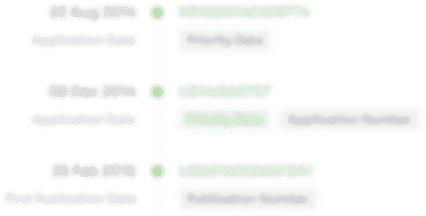
- R&D Engineer
- R&D Manager
- IP Professional
- Industry Leading Data Capabilities
- Powerful AI technology
- Patent DNA Extraction
Browse by: Latest US Patents, China's latest patents, Technical Efficacy Thesaurus, Application Domain, Technology Topic, Popular Technical Reports.
© 2024 PatSnap. All rights reserved.Legal|Privacy policy|Modern Slavery Act Transparency Statement|Sitemap|About US| Contact US: help@patsnap.com