Network traffic classification method and system based on deep learning, and electronic equipment
A network traffic and deep learning technology, applied in the field of network traffic classification, can solve the problems of little research and mining of local characteristics of network traffic original data, unstable classification performance, etc., to ensure high precision and high performance, improve classification efficiency, The effect of shortening training time
- Summary
- Abstract
- Description
- Claims
- Application Information
AI Technical Summary
Problems solved by technology
Method used
Image
Examples
Embodiment Construction
[0045] In order to make the purpose, technical solution and advantages of the present application clearer, the present application will be further described in detail below in conjunction with the accompanying drawings and embodiments. It should be understood that the specific embodiments described here are only used to explain the present application, not to limit the present application.
[0046] Aiming at the technical problems existing in the existing network traffic classification methods, the network traffic classification method based on deep learning in the embodiment of the present application uses the deep learning hidden feature extraction technology to accurately mine a large number of hidden traffic feature sets in network traffic, ensuring that network traffic classification In the process, the traffic feature set in the network traffic is fully and efficiently used to accurately classify and identify the network traffic.
[0047] Specifically, see figure 1 , is...
PUM
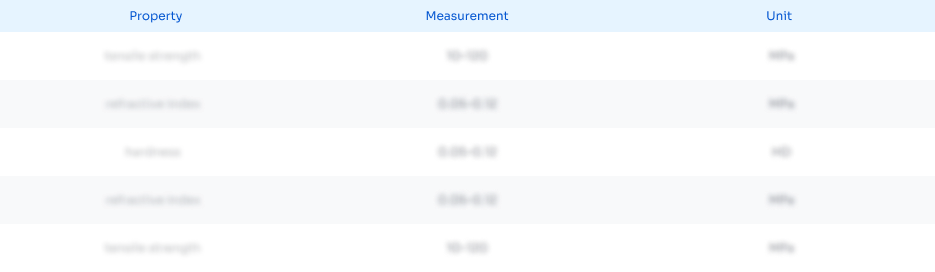
Abstract
Description
Claims
Application Information
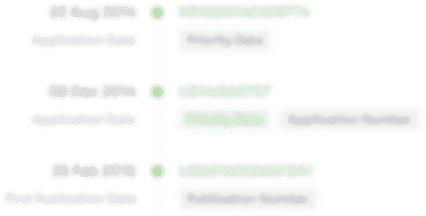
- R&D Engineer
- R&D Manager
- IP Professional
- Industry Leading Data Capabilities
- Powerful AI technology
- Patent DNA Extraction
Browse by: Latest US Patents, China's latest patents, Technical Efficacy Thesaurus, Application Domain, Technology Topic, Popular Technical Reports.
© 2024 PatSnap. All rights reserved.Legal|Privacy policy|Modern Slavery Act Transparency Statement|Sitemap|About US| Contact US: help@patsnap.com