Drift suppression method for electronic nose of domain self-adaptive extreme learning machine (ELM) based on domain correction
An extreme learning machine, domain adaptive technology, applied in scientific instruments, computer parts, measuring devices, etc., can solve the problem that the pattern recognition model cannot be directly applied to the electronic nose system.
- Summary
- Abstract
- Description
- Claims
- Application Information
AI Technical Summary
Problems solved by technology
Method used
Image
Examples
Embodiment Construction
[0024] The preferred embodiments of the present invention will be described in detail below with reference to the accompanying drawings.
[0025] The invention provides a domain-adaptive extreme learning machine drift suppression method based on domain correction. The method analyzes and solves the electronic nose drift problem from the perspective of data distribution. When drift occurs, the characteristic distribution of the data collected by the electronic nose at different times changes. If the model is trained with the data before the sensor drift, and then tested on the data after the sensor drift, this will not meet the assumption that the traditional machine learning requires the same distribution of data, thus degrading the prediction performance. Most of the current pattern recognition methods are trained from source domain data and their labels, and target domain samples with a considerable amount of information are often ignored.
[0026] After the method provided...
PUM
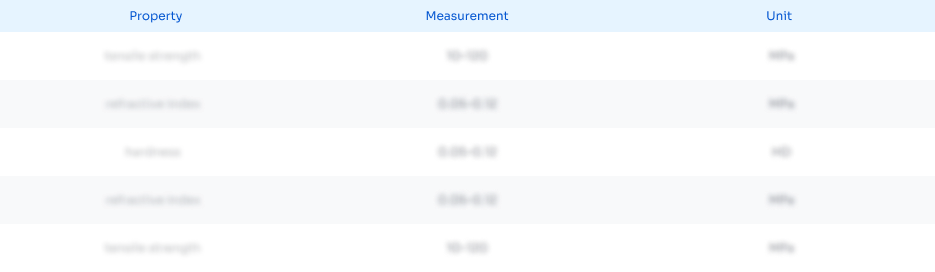
Abstract
Description
Claims
Application Information
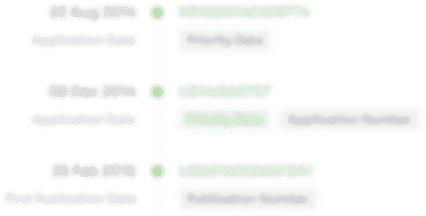
- R&D Engineer
- R&D Manager
- IP Professional
- Industry Leading Data Capabilities
- Powerful AI technology
- Patent DNA Extraction
Browse by: Latest US Patents, China's latest patents, Technical Efficacy Thesaurus, Application Domain, Technology Topic, Popular Technical Reports.
© 2024 PatSnap. All rights reserved.Legal|Privacy policy|Modern Slavery Act Transparency Statement|Sitemap|About US| Contact US: help@patsnap.com