An image quality evaluation method based on importance between deep network features
An image quality assessment, deep network technology, applied in the field of image quality assessment based on the importance of deep network features
- Summary
- Abstract
- Description
- Claims
- Application Information
AI Technical Summary
Problems solved by technology
Method used
Image
Examples
Embodiment Construction
[0030] Whole flow chart of the present invention is attached figure 1 Shown, the present invention is elaborated below in conjunction with accompanying drawing:
[0031] Step 1: Dataset Preparation
[0032]The image quality evaluation dataset is randomly divided into training set and test set according to the image content. The present invention selects four public IQA data sets, which are LIVE (including 779 distorted images, 5 types of distortion), TID2013 (including 3000 distorted images, 24 types of distortion), CSIQ (including 866 distorted images, 6 kinds of distorted images), LIVEMD (including 450 pairs of distorted images, 2 kinds of distortion types), and divide them according to the content of the original reference images in each database. The distorted images corresponding to 80% of the content are training sets, and the remaining 20% correspond to The distortion map of is the test set. During the specific implementation, the training set and test set in each ...
PUM
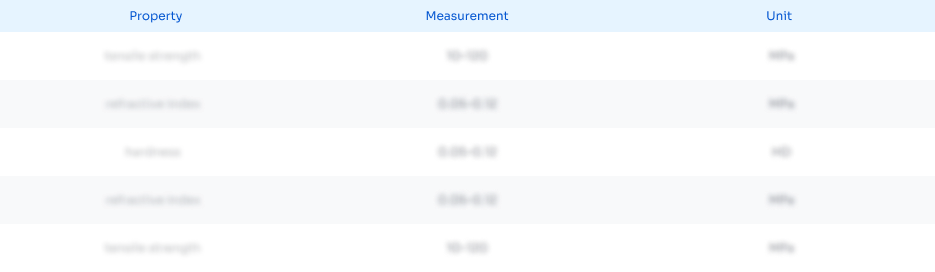
Abstract
Description
Claims
Application Information
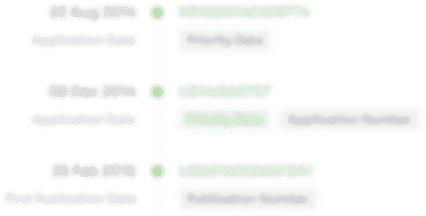
- R&D Engineer
- R&D Manager
- IP Professional
- Industry Leading Data Capabilities
- Powerful AI technology
- Patent DNA Extraction
Browse by: Latest US Patents, China's latest patents, Technical Efficacy Thesaurus, Application Domain, Technology Topic, Popular Technical Reports.
© 2024 PatSnap. All rights reserved.Legal|Privacy policy|Modern Slavery Act Transparency Statement|Sitemap|About US| Contact US: help@patsnap.com