A semi-supervised classification method for improving a generative adversarial network based on principal component analysis
A technology of principal component analysis and classification method, which is applied in the field of generative confrontation network improvement, can solve the problems of difficult training of generator parameters, high cost, and infeasibility, and achieve the goal of reducing the number of network iterations, improving classification accuracy, and improving accuracy Effect
- Summary
- Abstract
- Description
- Claims
- Application Information
AI Technical Summary
Problems solved by technology
Method used
Image
Examples
Embodiment Construction
[0055] In order to make the object, technical solution and advantages of the present invention more clear, the present invention will be further described in detail below in conjunction with the examples. It should be understood that the specific embodiments described here are only used to explain the present invention, not to limit the present invention.
[0056] The input of the generator in the generative confrontation network is random noise, and the randomness will make the parameters of the generator difficult to train, and it is difficult to generate high-quality pictures.
[0057] The generator and the discriminator are a mutual game process. Using the generative confrontation network for semi-supervised classification is to slightly adjust the output of the discriminator and change it to a classifier. The effect of the generator will affect the final discriminator (classifier). classification accuracy.
[0058] In order to solve the above technical problems, the pres...
PUM
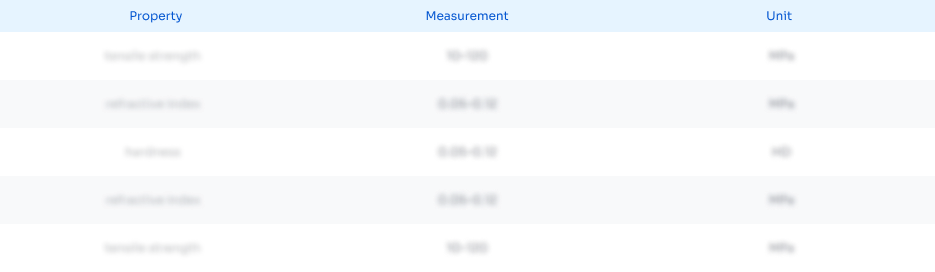
Abstract
Description
Claims
Application Information
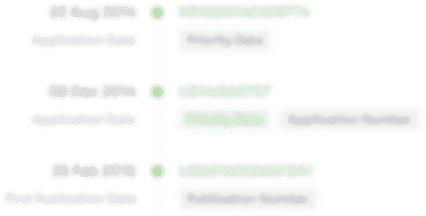
- R&D Engineer
- R&D Manager
- IP Professional
- Industry Leading Data Capabilities
- Powerful AI technology
- Patent DNA Extraction
Browse by: Latest US Patents, China's latest patents, Technical Efficacy Thesaurus, Application Domain, Technology Topic, Popular Technical Reports.
© 2024 PatSnap. All rights reserved.Legal|Privacy policy|Modern Slavery Act Transparency Statement|Sitemap|About US| Contact US: help@patsnap.com