A high-speed rail turnout intelligent fault detection method based on hybrid deep learning
A fault detection and deep learning technology, which is applied to electrical equipment, measuring electricity, measuring devices, etc. for manipulating switches or line circuit breakers. , a large amount of label data, etc.
- Summary
- Abstract
- Description
- Claims
- Application Information
AI Technical Summary
Problems solved by technology
Method used
Image
Examples
Embodiment 1
[0052] Embodiment 1 of the present invention provides a high-speed rail turnout intelligent fault detection method based on hybrid deep learning, such as figure 1 As shown, the method includes the following steps:
[0053] S1: Use the deep noise reduction self-encoder to automatically extract the characteristics of the current curve data of each turnout, and obtain the unlabeled feature data;
[0054] In step S1, the normal action process of the turnout is generally divided into three periods: unlocking-converting-locking, and the corresponding action current curve of the turnout is formed according to the action of each period.
[0055] In step S1, the depth noise reduction self-encoder has a structure such as figure 2 As shown, on the basis of the autoencoder, in order to make the autoencoder learn a more robust low-dimensional representation of high-dimensional data, noise is introduced on the basis of the original input vector, and then the noisy high-dimensional The in...
Embodiment 2
[0087] In order to evaluate the effectiveness of the proposed fault detection model, the experiment selected S700K switch machine field switch action current curve data for fault detection model training and verification of the test process, and a total of 1200 unlabeled data were selected.
[0088] refer to Figure 7 , the specific process is as follows:
[0089] A. Data preprocessing and data set division: take the original data of all switch operating current curves under the same switch machine, and the operating current curve of the switch is as follows: Figure 8 As shown, the original data of the selected switch action current curves are sorted in chronological order, and normalized, and then the data set is selected by stratified sampling method, 3 / 4 is used as the training set, and the other 1 / 4 is used as the test set , to label the data of the test set combined with expert knowledge.
[0090] B. Automatic feature extraction: build a noise-reducing self-encoder for...
PUM
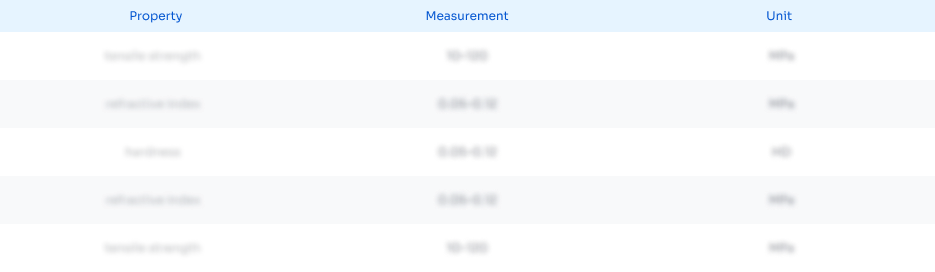
Abstract
Description
Claims
Application Information
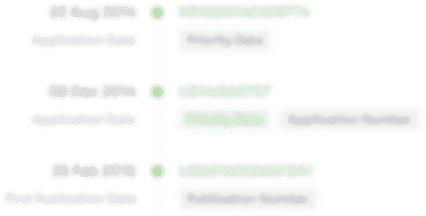
- R&D Engineer
- R&D Manager
- IP Professional
- Industry Leading Data Capabilities
- Powerful AI technology
- Patent DNA Extraction
Browse by: Latest US Patents, China's latest patents, Technical Efficacy Thesaurus, Application Domain, Technology Topic, Popular Technical Reports.
© 2024 PatSnap. All rights reserved.Legal|Privacy policy|Modern Slavery Act Transparency Statement|Sitemap|About US| Contact US: help@patsnap.com