Deep neural machine translation method based on dynamic linear aggregation
A deep neural and machine translation technology, applied in the field of machine translation, can solve the problems that the network structure cannot be effectively trained, the underlying network cannot be fully trained, and the translation performance cannot be further improved, so as to achieve robust training and improve information transmission. Efficiency, strong expressive effect
- Summary
- Abstract
- Description
- Claims
- Application Information
AI Technical Summary
Problems solved by technology
Method used
Image
Examples
Embodiment Construction
[0049] Researchers believe that different layers of networks in deep networks have different capabilities for feature extraction. Therefore, when calculating the upper layer network, the calculation results of the previous layer network should be effectively used, which will be more conducive to the feature learning of the upper layer network. Based on the above theory, the present invention adds a memory network to store the network output of the intermediate layer between the same layer at the encoding end and the decoding end of the Transformer model to form a Transformer model based on dynamic linear aggregation. Using the linear multi-step method in ordinary differential equations allows the current stacked network to make full use of the results of all previous stacked calculations to improve the efficiency of information transmission, so that the network can be stacked deeper and bring positive effects on performance. In the present invention, the adopted linear multi-st...
PUM
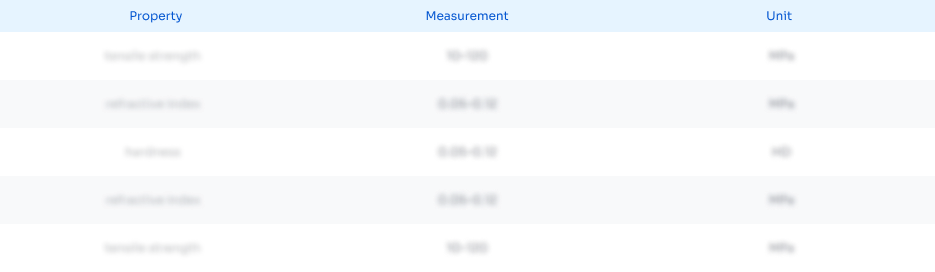
Abstract
Description
Claims
Application Information
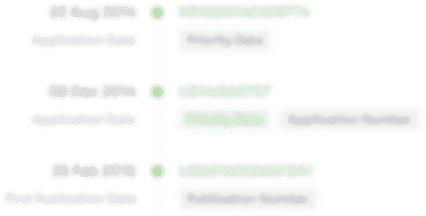
- R&D Engineer
- R&D Manager
- IP Professional
- Industry Leading Data Capabilities
- Powerful AI technology
- Patent DNA Extraction
Browse by: Latest US Patents, China's latest patents, Technical Efficacy Thesaurus, Application Domain, Technology Topic, Popular Technical Reports.
© 2024 PatSnap. All rights reserved.Legal|Privacy policy|Modern Slavery Act Transparency Statement|Sitemap|About US| Contact US: help@patsnap.com