An automatic driving scene key target detection and extraction method based on deep learning
A target detection and automatic driving technology, which is applied in the direction of instruments, biological neural network models, character and pattern recognition, etc., can solve the problem of unsatisfactory long-distance small target detection, lack of universal key target recognition framework, and the inability to achieve automatic target detection. Box selection and other issues, to achieve the effect of fast multi-target recognition, convenient experimental testing, and strong model generalization
- Summary
- Abstract
- Description
- Claims
- Application Information
AI Technical Summary
Problems solved by technology
Method used
Image
Examples
Embodiment Construction
[0053] The present invention will be described in detail below in conjunction with the accompanying drawings and specific embodiments. This embodiment is carried out on the premise of the technical solution of the present invention, and detailed implementation and specific operation process are given, but the protection scope of the present invention is not limited to the following embodiments.
[0054] The present invention provides a method for detecting and extracting key targets in automatic driving scenes based on deep learning. The method is based on an improved single shot multibox detector (SSD_ARC, Single Shot Multibox Detector on Aspect Ratiochanging) for key target detection and semantic annotation in driving scenes. And target frame selection, for driving scenarios, the detection accuracy is improved by optimizing the gradient update algorithm, learning rate reduction strategy and prior frame generation strategy.
[0055]1. SSD (Single Shot MultiBox Detector)
[0...
PUM
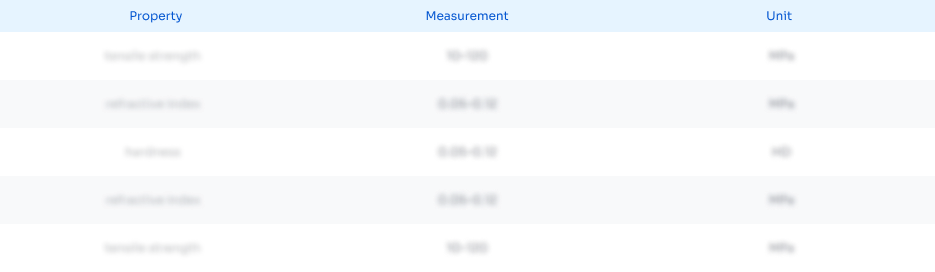
Abstract
Description
Claims
Application Information
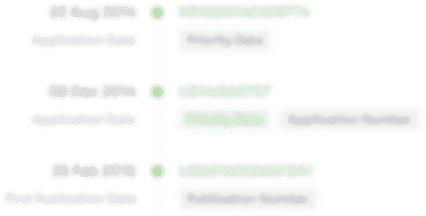
- R&D
- Intellectual Property
- Life Sciences
- Materials
- Tech Scout
- Unparalleled Data Quality
- Higher Quality Content
- 60% Fewer Hallucinations
Browse by: Latest US Patents, China's latest patents, Technical Efficacy Thesaurus, Application Domain, Technology Topic, Popular Technical Reports.
© 2025 PatSnap. All rights reserved.Legal|Privacy policy|Modern Slavery Act Transparency Statement|Sitemap|About US| Contact US: help@patsnap.com