An integrated real-time learning industrial process soft measurement modeling method based on multi-objective optimization
A multi-objective optimization and industrial process technology, applied in the field of integrated real-time learning soft sensor modeling based on multi-objective optimization, to achieve the effect of dealing with nonlinearity and improving computational efficiency
- Summary
- Abstract
- Description
- Claims
- Application Information
AI Technical Summary
Problems solved by technology
Method used
Image
Examples
Embodiment 1
[0028]Embodiment 1: as figure 1 As shown, an integrated real-time learning industrial process soft-sensing modeling method based on multi-objective optimization includes the following steps. The first step is to collect industrial process data through a distributed control system or offline detection method, and construct a soft-sensing modeling method for soft-sensing modeling database. Through the mechanism analysis of the industrial process, the auxiliary variables related to the predictor variables are determined.
[0029] Step 2: Normalize the samples in the database and divide them into training sets (D train ∈R J×Q ), validation set (D validate ∈R K×Q ) and the test set (D test ∈R T×Q ). The training set is used for model training, the validation set is used for model parameter optimization, and the test set is used for model performance evaluation.
[0030] The third step: use the multi-objective evolutionary optimization method (NSGA-II algorithm) to optimize ...
Embodiment 2
[0048] Example 2: The following will be further described in conjunction with the industrial process of the debutanizer. The debutanizer is a part of the device for desulfurization and naphtha separation in the industrial refining process, and its goal is to minimize the concentration of butane at the bottom of the tower. However, it is still difficult to realize real-time online detection of butane concentration at present. The on-line prediction of butane concentration by soft sensing method can effectively improve the desulfurization efficiency of debutanizer. According to the mechanism analysis, the x 1 Tower top temperature; x 2 pressure at the top of the tower; x 3 Return flow at the top of the tower; x 4 Outflow of top product; x 5 The sixth layer tray temperature; x 6 tower low temp 1;x 7 The seven monitoring variables of tower bottom temperature 2 are used as auxiliary variables to construct the soft sensor model, and the output variable is butane concentration....
PUM
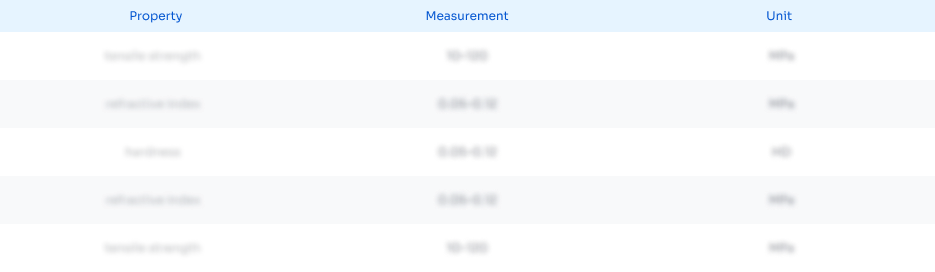
Abstract
Description
Claims
Application Information
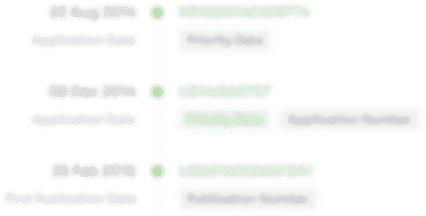
- R&D Engineer
- R&D Manager
- IP Professional
- Industry Leading Data Capabilities
- Powerful AI technology
- Patent DNA Extraction
Browse by: Latest US Patents, China's latest patents, Technical Efficacy Thesaurus, Application Domain, Technology Topic, Popular Technical Reports.
© 2024 PatSnap. All rights reserved.Legal|Privacy policy|Modern Slavery Act Transparency Statement|Sitemap|About US| Contact US: help@patsnap.com