A Method for Optimizing Random Forest Parameters for Machine Learning Model Training
A machine learning model and random forest technology, applied in biological models, computational models, instruments, etc., can solve problems such as unsuitable data sets, and achieve the effects of improving classification accuracy, obvious acceleration effect, and fast calculation speed.
- Summary
- Abstract
- Description
- Claims
- Application Information
AI Technical Summary
Problems solved by technology
Method used
Image
Examples
Embodiment Construction
[0027] In order to facilitate those of ordinary skill in the art to understand and implement the present invention, the present invention will be described in further detail below in conjunction with the accompanying drawings and embodiments. It should be understood that the implementation examples described here are only used to illustrate and explain the present invention, and are not intended to limit this invention.
[0028] please see figure 1 and figure 2 , a method for optimizing random forest parameters for machine learning model training provided by the invention, is characterized in that, comprises the following steps:
[0029] Step 1: Store the collected training set data in the HDFS distributed file system, and its storage path is the variable path. Taking LendingClub, a credit loan company in the United States as an example, its loan data can be obtained from the official website;
[0030] Step 2: Use the Antlion algorithm to perform global optimization on the ...
PUM
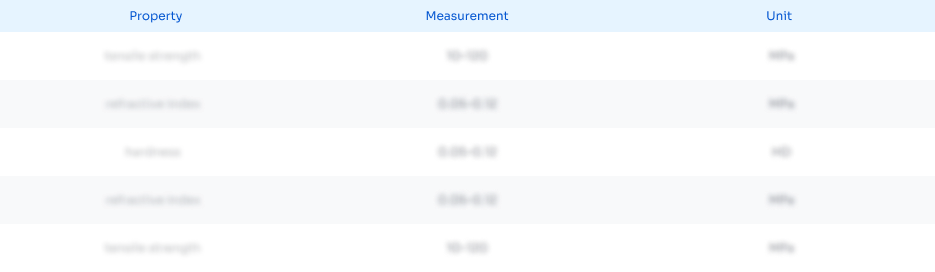
Abstract
Description
Claims
Application Information
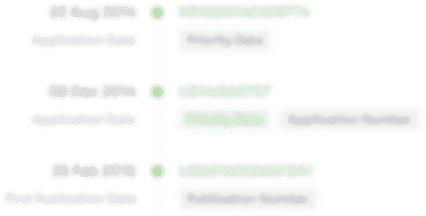
- R&D
- Intellectual Property
- Life Sciences
- Materials
- Tech Scout
- Unparalleled Data Quality
- Higher Quality Content
- 60% Fewer Hallucinations
Browse by: Latest US Patents, China's latest patents, Technical Efficacy Thesaurus, Application Domain, Technology Topic, Popular Technical Reports.
© 2025 PatSnap. All rights reserved.Legal|Privacy policy|Modern Slavery Act Transparency Statement|Sitemap|About US| Contact US: help@patsnap.com