A multi-modal medical image retrieval method based on multi-image regularization deep hashing
A medical image and multi-modal technology, applied in the field of medical image processing, can solve the problems that affect the retrieval accuracy, manual features cannot meet the high-precision retrieval requirements, and fail to maintain the local manifold structure.
- Summary
- Abstract
- Description
- Claims
- Application Information
AI Technical Summary
Problems solved by technology
Method used
Image
Examples
Embodiment Construction
[0039]The technical solutions in the embodiments of the present invention will be described clearly and in detail below with reference to the drawings in the embodiments of the present invention. The described embodiments are only some of the embodiments of the invention.
[0040] The technical scheme that the present invention solves the problems of the technologies described above is:
[0041] According to different data types of multimodal medical images, different types of depth models are selected for extracting their depth features, such as image data selection convolutional neural network, text can select cyclic neural network, etc., the model for extracting depth features is not the present invention key tasks.
[0042] Because the numerical dimensions of each feature are inconsistent, and the subsequent modality adaptive RBM model used for hash code learning requires the visual layer to conform to the Gaussian distribution, after extracting the depth features of the ...
PUM
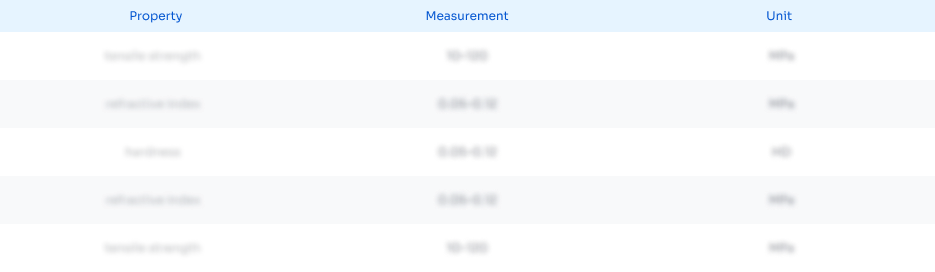
Abstract
Description
Claims
Application Information
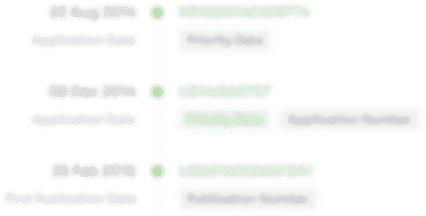
- Generate Ideas
- Intellectual Property
- Life Sciences
- Materials
- Tech Scout
- Unparalleled Data Quality
- Higher Quality Content
- 60% Fewer Hallucinations
Browse by: Latest US Patents, China's latest patents, Technical Efficacy Thesaurus, Application Domain, Technology Topic, Popular Technical Reports.
© 2025 PatSnap. All rights reserved.Legal|Privacy policy|Modern Slavery Act Transparency Statement|Sitemap|About US| Contact US: help@patsnap.com