A three-dimensional image segmentation method and system based on a full convolutional neural network
A convolutional neural network and three-dimensional image technology, applied in the field of three-dimensional sequence image segmentation, can solve the problems of poor robustness, multi-context information, incomplete structural information, etc., to achieve smooth output, accurate segmentation results, and optimized segmentation results.
- Summary
- Abstract
- Description
- Claims
- Application Information
AI Technical Summary
Problems solved by technology
Method used
Image
Examples
Embodiment
[0058] see figure 1 , a three-dimensional image segmentation method based on a fully convolutional neural network according to an embodiment of the present invention, applied to medical image processing and segmentation, includes the following steps:
[0059] S101 , acquiring the Abus ultrasound image and labeling the training data.
[0060] Abus breast screening ultrasound uses new technologies such as full-volume breast ultrasound imaging, contrast-enhanced ultrasound, elastography, and three-dimensional and four-dimensional imaging, and uses high-definition 3D volume images to provide high-homogeneity and high-resolution ultrasound images. The image format is DICOM, that is, digital medical imaging and communication, which is an international standard for medical images and related information; the image file contains many metadata information such as pixel size and picture size.
[0061] The scanned ultrasound image is a three-dimensional image according to the laws of th...
PUM
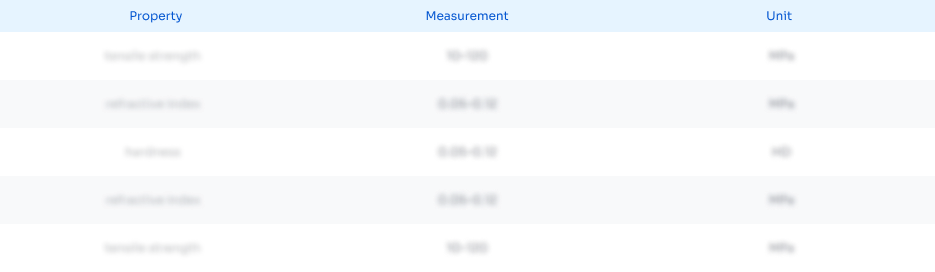
Abstract
Description
Claims
Application Information
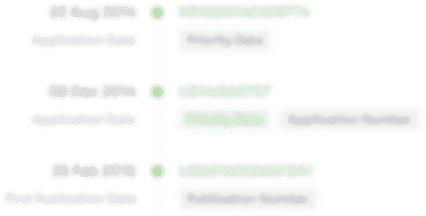
- R&D Engineer
- R&D Manager
- IP Professional
- Industry Leading Data Capabilities
- Powerful AI technology
- Patent DNA Extraction
Browse by: Latest US Patents, China's latest patents, Technical Efficacy Thesaurus, Application Domain, Technology Topic.
© 2024 PatSnap. All rights reserved.Legal|Privacy policy|Modern Slavery Act Transparency Statement|Sitemap