Deep learning model training method and device, training equipment and storage medium
A deep learning and model training technology, applied in the information field, can solve the problems of reduced training efficiency and difficulty in ensuring the processing accuracy of deep learning models
- Summary
- Abstract
- Description
- Claims
- Application Information
AI Technical Summary
Problems solved by technology
Method used
Image
Examples
example 1
[0330] The depth model provided in this example is a bottom-up deep learning model. This deep learning model is a two-step multi-target detection: In this example, the target is a human body as an example. For details, see Figure 5A with Figure 5B Shown:
[0331] The first step is to predict the first type of feature map and obtain the position of each key point; for example, the first type of feature map, the first feature map and / or the second feature map can be obtained by using a bottom-up key point model ;
[0332] The second step is to cluster the different features of each key point to obtain a complete human pose. In this example, an auxiliary training module can be used, for example, a clustering module based on human body posture guidance, respectively clustering the first features contained in the first feature map based on the first type feature map, and clustering the first features contained in the first feature map based on the first The class feature map c...
example 2
[0353] Such as Figure 6A As shown, this example provides a deep learning model, including:
[0354] Feature extraction layer, including: multiple convolutional sublayers and pooling layers, in Figure 6AThe number of convolutional sublayers in the middle is 5; the pooling layer is a maximum pooling layer, and the maximum pooling layer here is a downsampling layer that retains the maximum value; the channel number of the first convolutional sublayer is 64. The size of the convolution kernel is 7*7, and the convolution step is 2; the number of channels of the second convolution sublayer is 128, the size of the convolution kernel is 3*3, and the convolution step is 1; The number of channels of the three convolution sublayers is 128, the size of the convolution kernel is 7*7, and the convolution step is 1; the number of channels of the fourth convolution sublayer is 128, and the size of the convolution kernel is 3* 3. The convolution step is 1; the number of channels of the fif...
example 3
[0358] Human key point detection and tracking is the basis of video analysis, and has important application prospects in the field of security and motion analysis. The single-frame multi-person posture detection technology based on the first feature (Keypoint Embedding) is a very advanced bottom-up human key point detection technology.
[0359] This method outputs the first feature map while outputting the first feature map of the human body. The dimensionality of the embedded feature map and the first-class features Figure 1 It can also be represented by a series of two-dimensional matrices of output resolution size, where the category of each key point corresponds to a two-dimensional matrix. The first type of feature map and the embedded feature map can correspond one-to-one in the spatial position. In the output embedding feature map, each key point of the same person has similar embedding values, while the embedding values of key points of different people are very d...
PUM
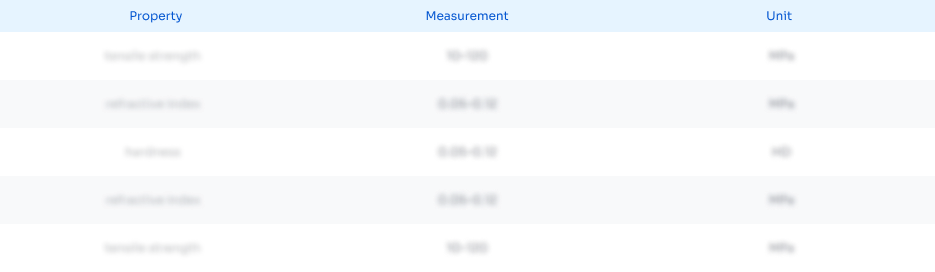
Abstract
Description
Claims
Application Information
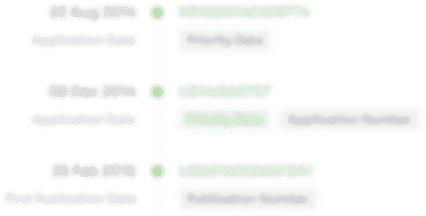
- R&D
- Intellectual Property
- Life Sciences
- Materials
- Tech Scout
- Unparalleled Data Quality
- Higher Quality Content
- 60% Fewer Hallucinations
Browse by: Latest US Patents, China's latest patents, Technical Efficacy Thesaurus, Application Domain, Technology Topic, Popular Technical Reports.
© 2025 PatSnap. All rights reserved.Legal|Privacy policy|Modern Slavery Act Transparency Statement|Sitemap|About US| Contact US: help@patsnap.com