Building extraction method based on gating depth residual error optimization network
An extraction method and building technology, applied in the field of computer vision, can solve problems such as roughness, easy to be ignored, environmental factors, etc., to increase diversity, avoid overfitting, and improve overall accuracy.
- Summary
- Abstract
- Description
- Claims
- Application Information
AI Technical Summary
Problems solved by technology
Method used
Image
Examples
Embodiment Construction
[0051] The following will clearly and completely describe the technical solutions in the embodiments of the present invention with reference to the accompanying drawings in the embodiments of the present invention. Obviously, the described embodiments are only some, not all, embodiments of the present invention. Based on the embodiments of the present invention, all other embodiments obtained by persons of ordinary skill in the art without creative efforts fall within the protection scope of the present invention.
[0052] The present invention involves a feature information gating transfer mechanism and a deep residual convolutional neural network. First, the feature combination of multi-source remote sensing data (including high-resolution aerial images and airborne LiDAR point cloud data) is obtained, and the The method increases the diversity of training samples, and then obtains the low-middle-high-level image features of the image through the improved deep residual encode...
PUM
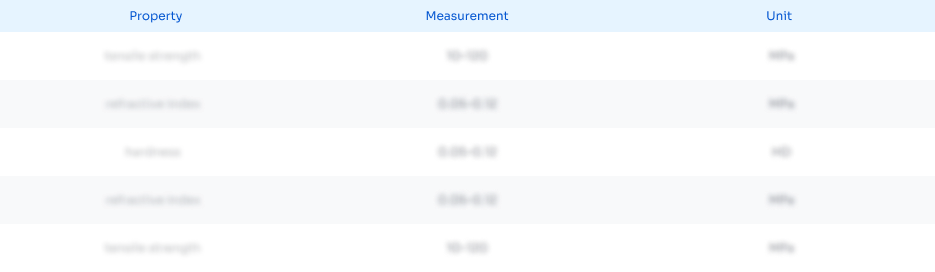
Abstract
Description
Claims
Application Information
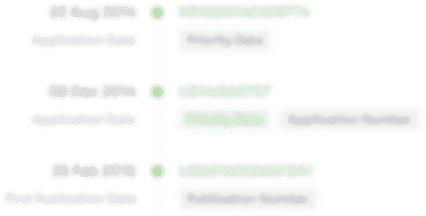
- Generate Ideas
- Intellectual Property
- Life Sciences
- Materials
- Tech Scout
- Unparalleled Data Quality
- Higher Quality Content
- 60% Fewer Hallucinations
Browse by: Latest US Patents, China's latest patents, Technical Efficacy Thesaurus, Application Domain, Technology Topic, Popular Technical Reports.
© 2025 PatSnap. All rights reserved.Legal|Privacy policy|Modern Slavery Act Transparency Statement|Sitemap|About US| Contact US: help@patsnap.com