Multi-intersection signal lamp cooperative control method based on deep reinforcement learning
A multi-intersection, collaborative control technology, applied in the traffic control system of road vehicles, traffic control system, traffic flow detection, etc., can solve the problems of low efficiency of reinforcement learning training, deep neural network consumption, large computing resources, etc., to achieve The effect of improving model accuracy and convergence speed, simple structure, and improving operating efficiency
- Summary
- Abstract
- Description
- Claims
- Application Information
AI Technical Summary
Problems solved by technology
Method used
Image
Examples
Embodiment 1
[0118]The model of the present invention combines two training methods, and the specific details are shown in Algorithm 1. When it needs to be emphasized, the new policy network in the reinforcement learning of the present invention is the same network as the policy network in the imitation learning. In training, first use imitation learning to imitate several times until the accuracy Acc reaches a threshold ξ, and then use reinforcement learning for further training. In the model corresponding to the single intersection experimental environment of the present invention, ξ=0.9 is taken. In the multi-intersection environment, since the expert strategy adopted in the present invention does not consider the coordination of multi-intersections, the present invention sets ξ=0.7 to encourage exploration.
[0119] Algorithm 1 A signal light control model that combines imitation learning and reinforcement learning
[0120] Initialize a new policy network π′ and old policy network π ...
PUM
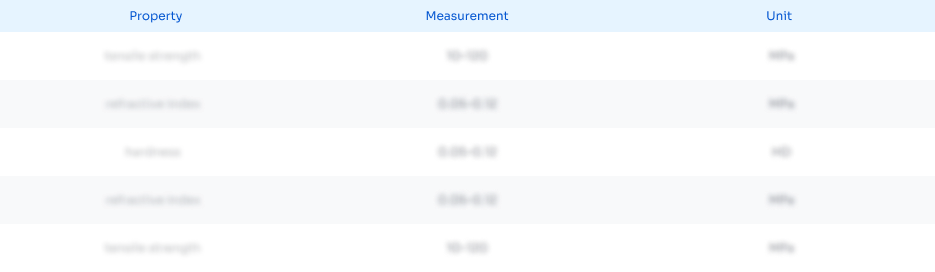
Abstract
Description
Claims
Application Information
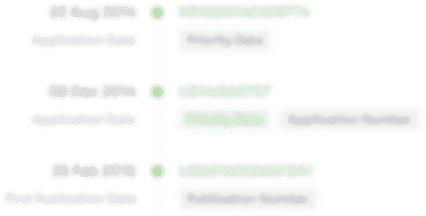
- R&D Engineer
- R&D Manager
- IP Professional
- Industry Leading Data Capabilities
- Powerful AI technology
- Patent DNA Extraction
Browse by: Latest US Patents, China's latest patents, Technical Efficacy Thesaurus, Application Domain, Technology Topic, Popular Technical Reports.
© 2024 PatSnap. All rights reserved.Legal|Privacy policy|Modern Slavery Act Transparency Statement|Sitemap|About US| Contact US: help@patsnap.com