Dangerous behavior automatic identification method based on double-flow convolutional neural network
A convolutional neural network, automatic recognition technology, applied in the field of human pose estimation and behavior recognition, can solve the problems of small video data set, inability to effectively extract continuous frame associations, and low quality.
- Summary
- Abstract
- Description
- Claims
- Application Information
AI Technical Summary
Problems solved by technology
Method used
Image
Examples
Embodiment Construction
[0045] Taking the automatic recognition of fighting actions as an example, the specific implementation is as follows:
[0046] Hardware environment:
[0047] The processing platform is AMAX's PSC-HB1X deep learning workstation, the processor is Inter(R) E5-2600 v3, the main frequency is 2.1GHZ, the memory is 128GB, the hard disk size is 1TB, and the graphics card model is GeForce GTX Titan X.
[0048] Software Environment:
[0049] Operating system Windows 10 64-bit; deep learning framework Tensorflow 1.1.0; integrated development environment python 3+Pycharm 2018.2.4 x64.
[0050] A method for automatic identification of dangerous behaviors based on a double-stream convolutional neural network provided by the present invention comprises the following steps:
[0051] Step1 Raw data preparation
[0052] Aiming at the common dangerous behaviors of people, three relatively typical dangerous behaviors of people, such as suicide, stealing and fighting, were selected, and a total...
PUM
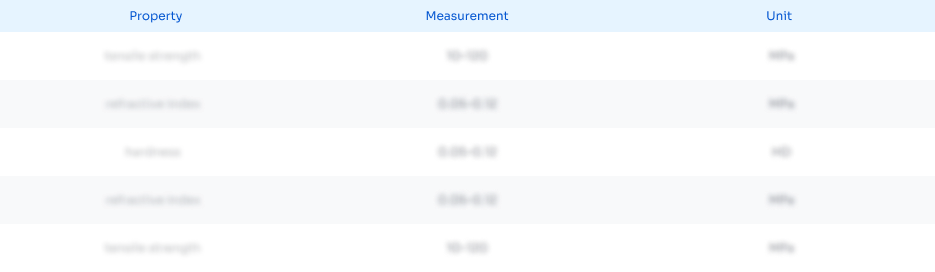
Abstract
Description
Claims
Application Information
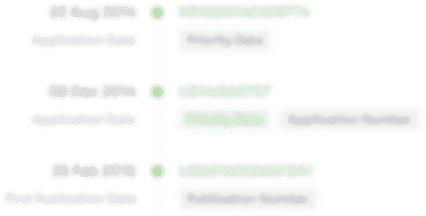
- R&D Engineer
- R&D Manager
- IP Professional
- Industry Leading Data Capabilities
- Powerful AI technology
- Patent DNA Extraction
Browse by: Latest US Patents, China's latest patents, Technical Efficacy Thesaurus, Application Domain, Technology Topic.
© 2024 PatSnap. All rights reserved.Legal|Privacy policy|Modern Slavery Act Transparency Statement|Sitemap