Multichannel LSTM neural network influenza epidemic situation prediction method based on attention mechanism
A neural network and prediction method technology, applied in the field of influenza epidemic prediction, can solve the problems of low prediction accuracy of influenza epidemic prediction technology, achieve accurate and effective real-time prediction, solve the problem of influenza prediction, and improve the effect of accuracy
- Summary
- Abstract
- Description
- Claims
- Application Information
AI Technical Summary
Problems solved by technology
Method used
Image
Examples
specific Embodiment approach 1
[0024] Specific implementation mode one: combine figure 1 The present embodiment is described, and the multi-channel LSTM neural network influenza epidemic prediction method based on the attention mechanism that the present embodiment provides specifically includes the following steps:
[0025] Step 1. Preprocessing and normalizing the data in the data set; then using model-based ranking for feature selection, and dividing the selected data into weather-related data and influenza epidemic-related data Two categories, generate a training set;
[0026] Step 2. Establish a multi-channel LSTM neural network model including attention mechanism (Attention); here the model is named Att-MCLSTM, namely Attention-based multi-channel LSTM (multi-channel LSTM neural network based on attention mechanism). The multi-channel LSTM neural network model based on the attention mechanism is different from the traditional recurrent neural network RNN (Recurrent Neural Network), and the LSTM neu...
specific Embodiment approach 2
[0032] Embodiment 2: The difference between this embodiment and Embodiment 1 is that the standardization described in Step 1 specifically includes the following processes:
[0033] Perform Min-Max normalization on the preprocessed data (also known as dispersion standardization):
[0034]
[0035] Among them, x is the value in the preprocessed data, x min is the minimum value in the preprocessed data, x max is the maximum value in the preprocessed data, and y is the value of x after Min-Max normalization processing; after data normalization, the data values will be scaled between 0 and 1.
[0036] Other steps and parameters are the same as those in the first embodiment.
specific Embodiment approach 3
[0037] Specific implementation mode three: the difference between this implementation mode and specific implementation mode two is that, as figure 2 As shown, the LSTM memory unit in the multi-channel LSTM neural network model described in step 2 includes an input gate 1, an output gate 2, a forgetting gate 3 and a self-circulating neuron 4; the structure gate structure of the LSTM memory unit controls the data in the LSTM The transmission in the memory unit includes the data transmission between different units and the data transmission within the unit. The input gate 1 controls the state update process of the unit, the output gate 2 controls whether the output sequence of the unit will change the memory state of other units, and the forgetting gate 3 can selectively retain or forget the previous state.
[0038] LSTM memory cells can be represented by the following equations:
[0039]
[0040] Among them, σ(·) is a logistic sigmoid function (mapping variables between 0 a...
PUM
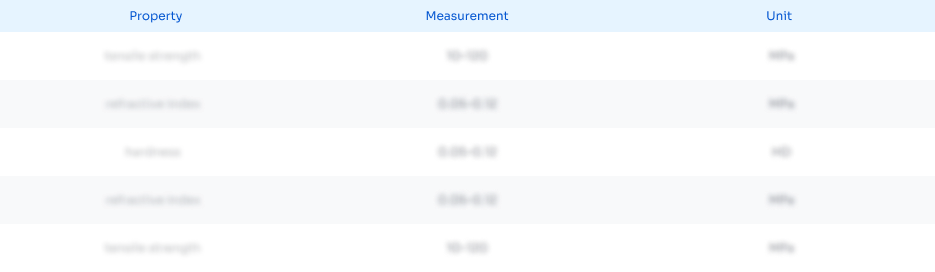
Abstract
Description
Claims
Application Information
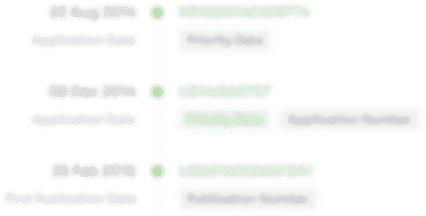
- R&D Engineer
- R&D Manager
- IP Professional
- Industry Leading Data Capabilities
- Powerful AI technology
- Patent DNA Extraction
Browse by: Latest US Patents, China's latest patents, Technical Efficacy Thesaurus, Application Domain, Technology Topic, Popular Technical Reports.
© 2024 PatSnap. All rights reserved.Legal|Privacy policy|Modern Slavery Act Transparency Statement|Sitemap|About US| Contact US: help@patsnap.com