A Few-Shot Polsar Image Classification Method Based on Fuzzy Label Semantic Prior
A classification method and small sample technology, applied in the field of image processing, can solve the problem of low classification accuracy of PolSAR, avoid the process of selecting and adjusting features, achieve fast training speed, and maintain consistency
- Summary
- Abstract
- Description
- Claims
- Application Information
AI Technical Summary
Problems solved by technology
Method used
Image
Examples
Embodiment Construction
[0066] The present invention provides a small-sample PolSAR image classification method based on the fuzzy label semantic prior, prepares the PolSAR image to be classified; takes the modulus of the coherence matrix T to obtain real-numbered network input data X; For training samples with label information, the sampling ratio of each category is 1%, and the sampling matrix A that records the position information of the training samples with label information and the sampling label matrix that records the pixel label information at the corresponding position are obtained Then use the sample label matrix Initialize the classification matrix Y; build a fully convolutional network FCN; then send the data set X into the built FCN, and use the sampled supervision information and the classification matrix Y to train the FCN; output the trained FCN prediction result Y FCN , each element represents the probability that the pixel is divided into each category; then use the prediction...
PUM
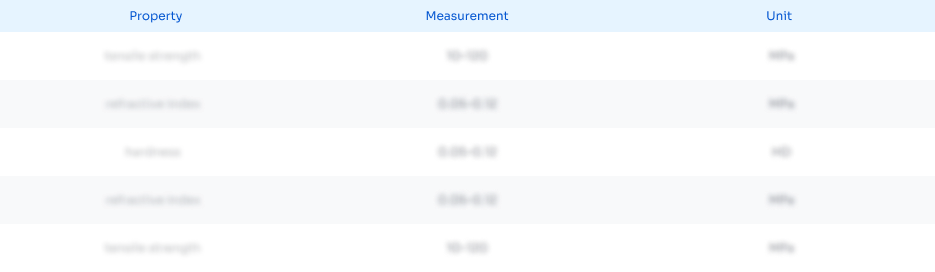
Abstract
Description
Claims
Application Information
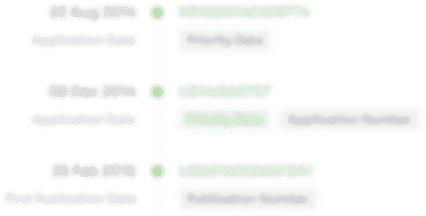
- R&D Engineer
- R&D Manager
- IP Professional
- Industry Leading Data Capabilities
- Powerful AI technology
- Patent DNA Extraction
Browse by: Latest US Patents, China's latest patents, Technical Efficacy Thesaurus, Application Domain, Technology Topic.
© 2024 PatSnap. All rights reserved.Legal|Privacy policy|Modern Slavery Act Transparency Statement|Sitemap