Load integration prediction method based on CNN-SVR model
A forecasting method and load forecasting technology, applied in forecasting, neural learning methods, biological neural network models, etc., can solve the problems of unanalyzed user load forecasting, unanalyzed and other problems, so as to improve the overall load forecasting accuracy, long training time, difficult effect
- Summary
- Abstract
- Description
- Claims
- Application Information
AI Technical Summary
Problems solved by technology
Method used
Image
Examples
Embodiment Construction
[0036] The present invention will be described in detail below in conjunction with the accompanying drawings and specific embodiments. Apparently, the described embodiments are some, not all, embodiments of the present invention. Based on the embodiments of the present invention, all other embodiments obtained by persons of ordinary skill in the art without making creative efforts shall fall within the protection scope of the present invention.
[0037] Such as figure 1 As shown, the present invention relates to a kind of load integrated prediction method based on CNN-SVR model, specifically comprises the following steps:
[0038] S1. Initialize the daily high and low temperature, climate, date type and other influencing factors and the daily load data of all users in a certain area, and set the number of users in a certain area as K bits.
[0039] S2. Calculate the monthly load, monthly load average, median, standard deviation, and Pearson correlation coefficient between th...
PUM
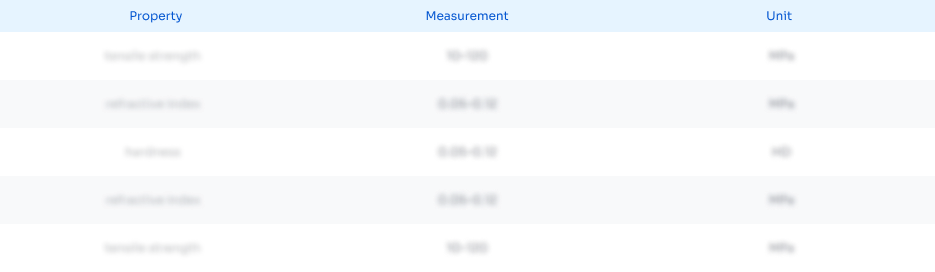
Abstract
Description
Claims
Application Information
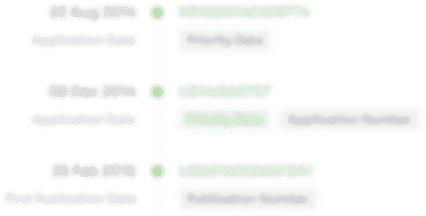
- R&D Engineer
- R&D Manager
- IP Professional
- Industry Leading Data Capabilities
- Powerful AI technology
- Patent DNA Extraction
Browse by: Latest US Patents, China's latest patents, Technical Efficacy Thesaurus, Application Domain, Technology Topic, Popular Technical Reports.
© 2024 PatSnap. All rights reserved.Legal|Privacy policy|Modern Slavery Act Transparency Statement|Sitemap|About US| Contact US: help@patsnap.com