An improved method of approximate information transfer algorithm based on deep learning denoising
An information transfer and deep learning technology, applied in computing, image enhancement, image analysis and other directions, can solve problems such as rate-distortion performance gap, and achieve the effect of enhancing matching degree, expanding applicability, and good denoising effect.
- Summary
- Abstract
- Description
- Claims
- Application Information
AI Technical Summary
Problems solved by technology
Method used
Image
Examples
Embodiment Construction
[0045] The present invention provides an enhancement method based on the approximate information transfer algorithm (LDAMP) of deep learning denoising. Firstly, through the detailed stratification of the noise level, the optimized denoising model is used to fully learn the noise of each level Then replace the denoising model in the LDAMP method with the trained denoising model, and then iterate according to the iterative strategy in DAMP, finally get the reconstructed image of the measured value, and achieve the reconstruction image quality compared with LDAMP at the same sampling rate obvious improvement. The effectiveness of this scheme can also be seen from the experimental link. It provides an effective idea to solve the problem of high-quality restoration of compressed sensing images under low sampling rate under conditions in reality.
[0046] see figure 1 , the present invention a kind of enhancement method based on the approximation information transfer algorithm (LD...
PUM
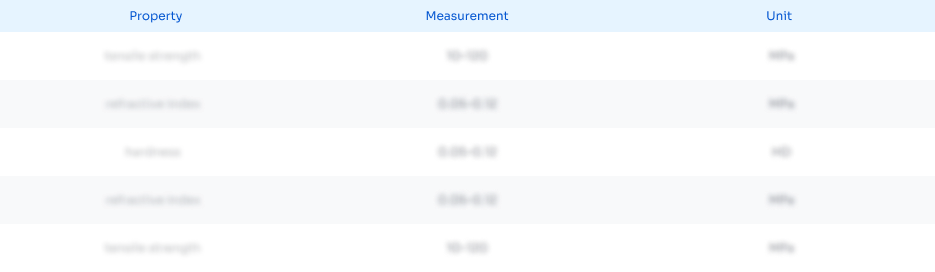
Abstract
Description
Claims
Application Information
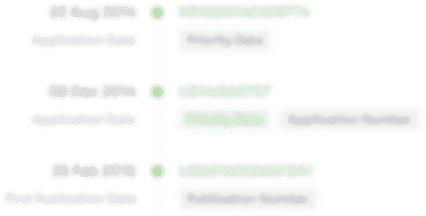
- R&D Engineer
- R&D Manager
- IP Professional
- Industry Leading Data Capabilities
- Powerful AI technology
- Patent DNA Extraction
Browse by: Latest US Patents, China's latest patents, Technical Efficacy Thesaurus, Application Domain, Technology Topic.
© 2024 PatSnap. All rights reserved.Legal|Privacy policy|Modern Slavery Act Transparency Statement|Sitemap