Multi-target deep belief network for mid-term power load predictions
A deep belief network, power load technology, applied in forecasting, biological neural network model, data processing applications, etc., can solve problems such as increased error and increased workload
- Summary
- Abstract
- Description
- Claims
- Application Information
AI Technical Summary
Problems solved by technology
Method used
Image
Examples
Embodiment Construction
[0019] Firstly, the collected power load data is processed, and the present invention utilizes the following formula to standardize the data:
[0020]
[0021] The data used in the present invention is collected once every half hour, and there will be 48 data every day. Using the time window method, every 48 data sets are taken as a group, and the next set of data is always separated from the previous set of data by half an hour—that is, the time window moves by half an hour. After using the time window, an n×m matrix will be generated, where the row vector n is the number of samples, the column vector m is the sample dimension, and m is the length of the time window 48. The empirical mode decomposition (EMD) is used to decompose the data, the decomposed data and the original data are integrated into a data set, and the data set is divided into a training set and a test set.
[0022] according to Figure II Describe the implementation steps of the algorithm:
[0023] Ste...
PUM
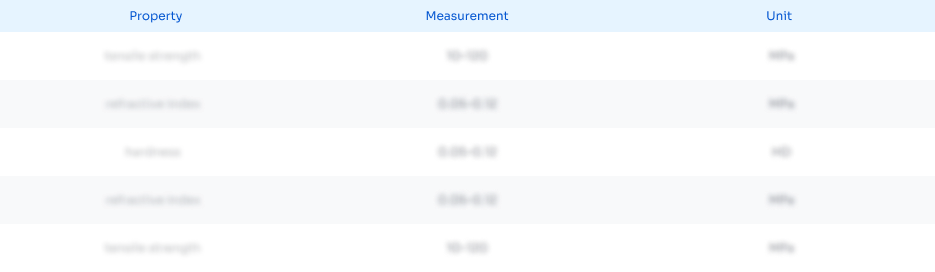
Abstract
Description
Claims
Application Information
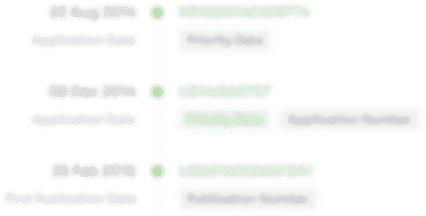
- R&D Engineer
- R&D Manager
- IP Professional
- Industry Leading Data Capabilities
- Powerful AI technology
- Patent DNA Extraction
Browse by: Latest US Patents, China's latest patents, Technical Efficacy Thesaurus, Application Domain, Technology Topic, Popular Technical Reports.
© 2024 PatSnap. All rights reserved.Legal|Privacy policy|Modern Slavery Act Transparency Statement|Sitemap|About US| Contact US: help@patsnap.com