Micro-seismic signal classification and identification method based on deep learning
A signal classification and deep learning technology, applied in the field of microseismic signal classification and identification based on deep learning, can solve problems such as EMD decomposition signal instability, affecting signal classification and identification accuracy, and poor microseismic signal analysis effect, etc., to achieve good technical value and application prospects, strong adaptability and real-time performance, and simple algorithm
- Summary
- Abstract
- Description
- Claims
- Application Information
AI Technical Summary
Problems solved by technology
Method used
Image
Examples
Embodiment Construction
[0053] The present invention proposes a method for classifying and identifying microseismic signals based on deep learning. In order to make the advantages and technical solutions of the present invention clearer and clearer, the present invention will be described in detail below in conjunction with specific embodiments.
[0054]A method for classification and identification of microseismic signals based on deep learning, the process is as follows figure 1 As shown, it specifically includes the following steps:
[0055] Step 1: Select M coal and rock fracture microseismic signals and N blasting vibration signals to form sample data sets of two types of vibration signals;
[0056] Step 2: Extract the main frequency F of M coal-rock fracture microseismic signals and N blasting vibration signals m , post-peak attenuation coefficient b, energy center of gravity coefficient C x Constitute the sample feature data training set and test set;
[0057] Further, in step 2, the main f...
PUM
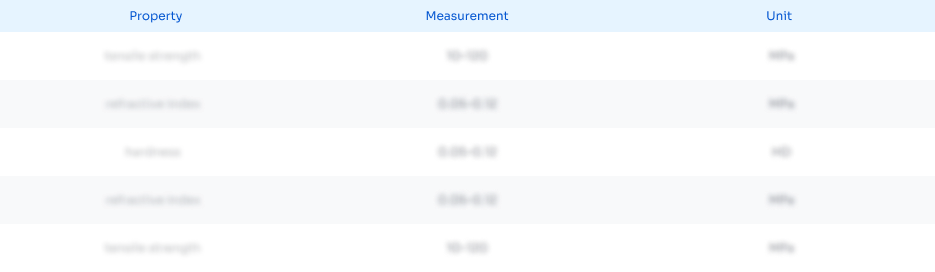
Abstract
Description
Claims
Application Information
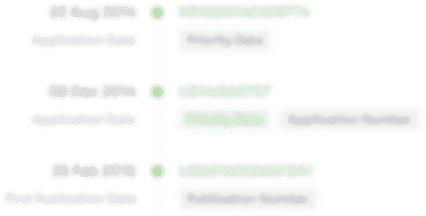
- R&D
- Intellectual Property
- Life Sciences
- Materials
- Tech Scout
- Unparalleled Data Quality
- Higher Quality Content
- 60% Fewer Hallucinations
Browse by: Latest US Patents, China's latest patents, Technical Efficacy Thesaurus, Application Domain, Technology Topic, Popular Technical Reports.
© 2025 PatSnap. All rights reserved.Legal|Privacy policy|Modern Slavery Act Transparency Statement|Sitemap|About US| Contact US: help@patsnap.com