Assembly robot part deep learning recognition method
A deep learning and recognition method technology, applied in the field of parts recognition, can solve the problems of lack of robustness, easy to miss detection and false detection, and achieve the effect of high recognition accuracy and good detection effect.
- Summary
- Abstract
- Description
- Claims
- Application Information
AI Technical Summary
Problems solved by technology
Method used
Image
Examples
Embodiment Construction
[0034] The present invention will be described in further detail below in conjunction with embodiment.
[0035] This embodiment is based on the YOLOv3 algorithm, and optimizes and improves the feature extraction network structure for part detection in the machine vision system. The parts have a good detection effect. The whole identification process is as follows figure 1 shown.
[0036] 1. The principle of real-time part recognition based on YOLOv3
[0037] YOLOv3 designed a new basic classification network Darknet-53 based on the Darknet-19 network structure in ResNet and YOLOv2, which contains a total of 53 convolutional layers. Only 1x1 and 3x3 small convolution kernels are used in the network, and more filters are generated while reducing parameters, so as to obtain a more distinguishable mapping function and reduce the possibility of overfitting; use a step size of 2 The convolution kernel replaces the pooling layer for dimensionality reduction operations to maintain...
PUM
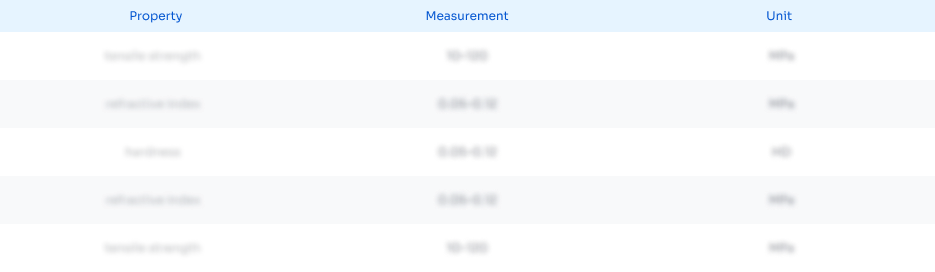
Abstract
Description
Claims
Application Information
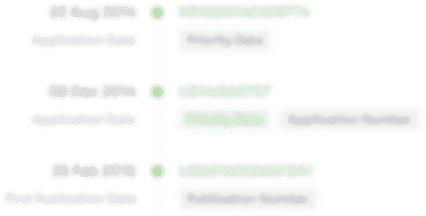
- R&D
- Intellectual Property
- Life Sciences
- Materials
- Tech Scout
- Unparalleled Data Quality
- Higher Quality Content
- 60% Fewer Hallucinations
Browse by: Latest US Patents, China's latest patents, Technical Efficacy Thesaurus, Application Domain, Technology Topic, Popular Technical Reports.
© 2025 PatSnap. All rights reserved.Legal|Privacy policy|Modern Slavery Act Transparency Statement|Sitemap|About US| Contact US: help@patsnap.com