Monocular light field image unsupervised depth estimation method based on convolutional neural network
A convolutional neural network and depth estimation technology, which is applied in the field of unsupervised depth estimation of monocular field images, which can solve the problem of accurate labeling difficulties, and achieve the effect of accurate, fast and efficient image depth estimation results.
- Summary
- Abstract
- Description
- Claims
- Application Information
AI Technical Summary
Problems solved by technology
Method used
Image
Examples
Embodiment Construction
[0054] The present invention will be further described below in conjunction with drawings and embodiments.
[0055] Such as figure 1 As shown, the unsupervised depth estimation method for monocular field images based on convolutional neural network, specifically includes the following steps:
[0056] Step 1. The experimental data set of the present invention is based on the light field image data set disclosed by Stanford using the Lytroillum light field camera to shoot real objects in the real world. The data set includes a large number of plants, flowers, street scenes and some sculpture images. These images are preprocessed and enhanced. The enhancement methods mainly used in the present invention include enhancing image brightness, horizontal / vertical flipping and random cutting, etc. After image enhancement, the data set is further expanded, and training samples and test samples are increased. The diversity of the network model will be further enhanced, and the generali...
PUM
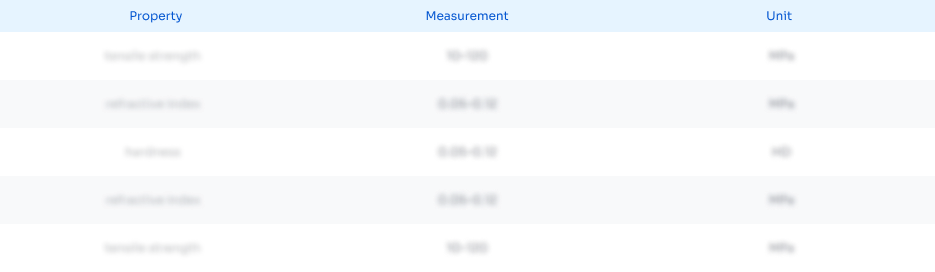
Abstract
Description
Claims
Application Information
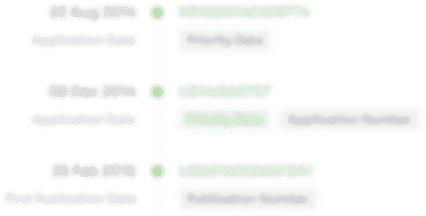
- R&D Engineer
- R&D Manager
- IP Professional
- Industry Leading Data Capabilities
- Powerful AI technology
- Patent DNA Extraction
Browse by: Latest US Patents, China's latest patents, Technical Efficacy Thesaurus, Application Domain, Technology Topic, Popular Technical Reports.
© 2024 PatSnap. All rights reserved.Legal|Privacy policy|Modern Slavery Act Transparency Statement|Sitemap|About US| Contact US: help@patsnap.com