Noise classifying method based on convolutional neural network
A convolutional neural network and noise classification technology, applied in the field of noise classification, can solve the problems of low noise recognition accuracy, poor statistical characteristics, and low noise classification accuracy, so as to reduce computational complexity, improve robustness, and improve The effect of accuracy
- Summary
- Abstract
- Description
- Claims
- Application Information
AI Technical Summary
Problems solved by technology
Method used
Image
Examples
specific example
[0063] Step 1: Preprocessing
[0064] The 15 types of noise signals in the Noisex-92 noise library are divided into frames and windowed. The frame length of each frame signal is 512 sampling points, and the window function uses Hamming window. Each continuous 12-frame signal is regarded as a noise sample, and 60,000 samples are selected as a training set, and 10,000 samples are used as a test set.
[0065] Step 2: Extract features
[0066] The 24-dimensional MFCC and ΔMFCC features are extracted from each frame signal in the sample, and the eigenvalues of a total of 12 frames of signals in each sample are combined into a two-dimensional matrix with a size of 12*24 as the time-frequency feature of each sample.
[0067] Step 3: Build the CNN structure
[0068] CNN network consists of input layer 1, first convolutional layer 2.1, first pooling layer 2.2, second convolutional layer 2.1, second pooling layer 2.2, fully connected layer 3 and output layer 4. Such as figure 1 sh...
PUM
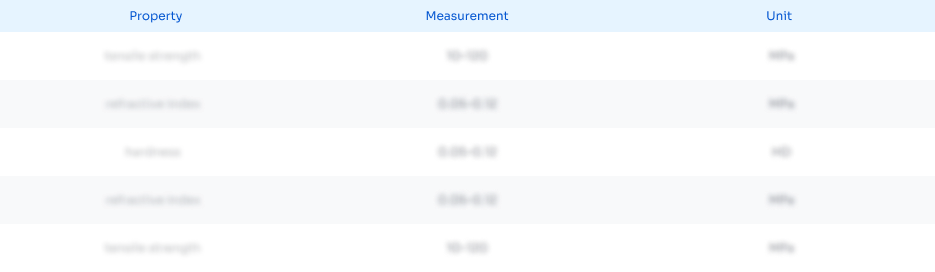
Abstract
Description
Claims
Application Information
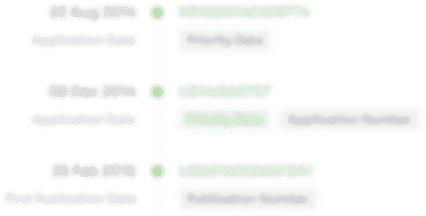
- R&D
- Intellectual Property
- Life Sciences
- Materials
- Tech Scout
- Unparalleled Data Quality
- Higher Quality Content
- 60% Fewer Hallucinations
Browse by: Latest US Patents, China's latest patents, Technical Efficacy Thesaurus, Application Domain, Technology Topic, Popular Technical Reports.
© 2025 PatSnap. All rights reserved.Legal|Privacy policy|Modern Slavery Act Transparency Statement|Sitemap|About US| Contact US: help@patsnap.com