Electric energy quality mixed disturbance analysis method based on deep learning
A technology of power quality and deep learning, applied in the direction of instruments, biological neural network models, data processing applications, etc., can solve problems such as poor recognition rate, lack of specific data, artificial neural network stop training, etc., to increase differentiation and improve The effect on classification ability
- Summary
- Abstract
- Description
- Claims
- Application Information
AI Technical Summary
Problems solved by technology
Method used
Image
Examples
Embodiment Construction
[0026] The present invention will be described in detail below with reference to the accompanying drawings and examples.
[0027] The present invention provides a power quality mixed disturbance analysis method based on deep learning. First, the time resolution and frequency resolution of the S transform are changed by adjusting the Gaussian window function decay rate, which is more conducive to the distinction of different types of power quality signals; and then A deep learning power quality disturbance analysis model based on the LSTM network structure in the feedback neural network RNN was constructed. Based on the more powerful feature analysis capabilities of the deep learning model, the disturbance type can be quickly and accurately identified.
[0028] Specifically include the following steps:
[0029] Step 1. Establish a power quality mixed interference signal training set, and build training based on seven types of power quality interference (voltage swell, voltage...
PUM
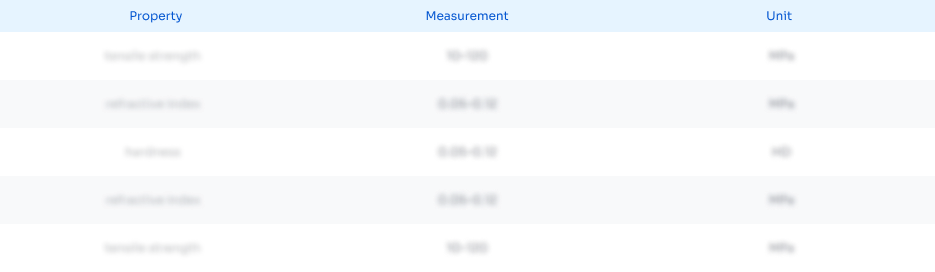
Abstract
Description
Claims
Application Information
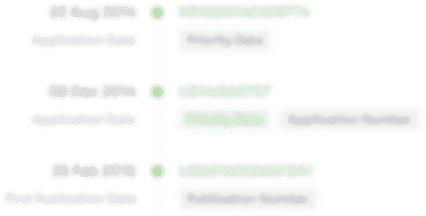
- R&D
- Intellectual Property
- Life Sciences
- Materials
- Tech Scout
- Unparalleled Data Quality
- Higher Quality Content
- 60% Fewer Hallucinations
Browse by: Latest US Patents, China's latest patents, Technical Efficacy Thesaurus, Application Domain, Technology Topic, Popular Technical Reports.
© 2025 PatSnap. All rights reserved.Legal|Privacy policy|Modern Slavery Act Transparency Statement|Sitemap|About US| Contact US: help@patsnap.com