A face noise dataset CNN training method based on the overall cosine distribution
A data set and training data set technology, applied in the field of image recognition, can solve the problems of difficult acquisition of prior knowledge, large fluctuation of loss value, poor recognition effect, etc., achieving small memory resources, large representation gains, and small computing resources. Effect
- Summary
- Abstract
- Description
- Claims
- Application Information
AI Technical Summary
Problems solved by technology
Method used
Image
Examples
Embodiment Construction
[0065] The present invention proposes a face noise data set CNN training method based on the overall cosine distribution, which will be further described in detail below in conjunction with the accompanying drawings and specific embodiments. The embodiments described in the present invention are exemplary, and are only used for explaining the present invention, but not construed as limiting the present invention.
[0066] The present invention proposes a kind of face noise data set CNN training method based on overall cosine distribution, comprises the following steps:
[0067] 1) Select the face training data set containing noise and record it as D all (the human face training data set comprises human face sample picture and the corresponding label of each picture, can adopt off-the-shelf human face training data set, wherein the number of label categories is not less than 1000, the number of human face sample pictures of each category Not less than 10), construct a benchmar...
PUM
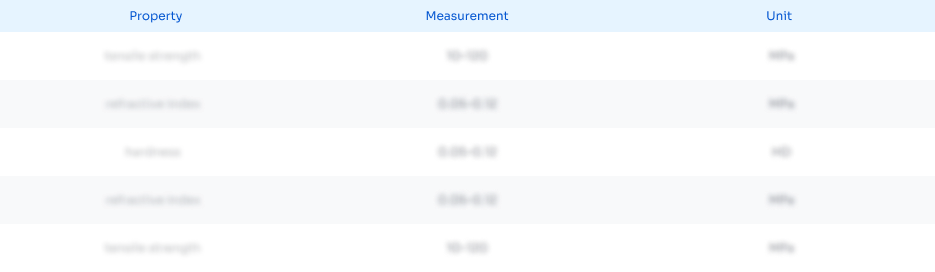
Abstract
Description
Claims
Application Information
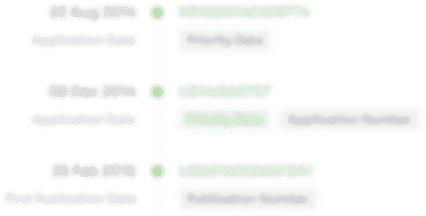
- R&D Engineer
- R&D Manager
- IP Professional
- Industry Leading Data Capabilities
- Powerful AI technology
- Patent DNA Extraction
Browse by: Latest US Patents, China's latest patents, Technical Efficacy Thesaurus, Application Domain, Technology Topic, Popular Technical Reports.
© 2024 PatSnap. All rights reserved.Legal|Privacy policy|Modern Slavery Act Transparency Statement|Sitemap|About US| Contact US: help@patsnap.com