Road blocking information extraction based on deep learning image semantic segmentation
A technology of semantic segmentation and deep learning, applied in image analysis, image enhancement, image data processing and other directions, can solve the problems of difficult to obtain road surface, increase in feature calculation, and inability to completely match the results of road vector extraction before disasters.
- Summary
- Abstract
- Description
- Claims
- Application Information
AI Technical Summary
Problems solved by technology
Method used
Image
Examples
Embodiment Construction
[0101] based on figure 1 The flow chart of the FCN-based road blockage information extraction technology shown in the figure is specifically introduced as follows.
[0102] 1. Sample vectorization method and sample library construction
[0103] 1. Vectorization of samples
[0104] Similar to the CNN model, the training of the FCN model requires a large number of samples. The difference is that generating the samples required for FCN model training requires first vectorizing the road on the image. Try to overcome the influence of trees and shadow occlusion when vectorizing the boundary of road samples, so as to help the convolutional neural network better distinguish the difference between the occlusion of trees and shadows on the road and the real road blocking, so as to deal with shadows and tree occlusions. The weakening of image road features and the misjudgment of non-blocking roads. After the road surface is vectorized, the road surface vector is converted into a raste...
PUM
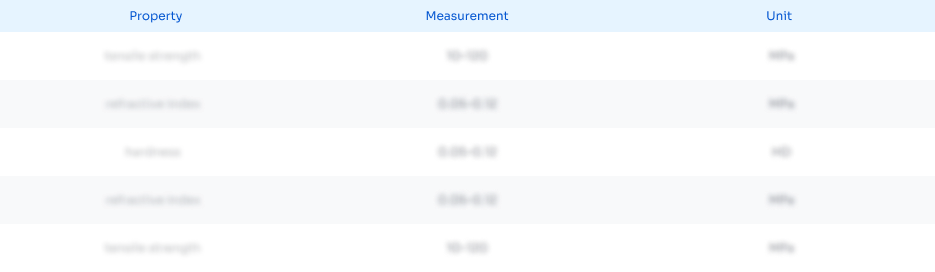
Abstract
Description
Claims
Application Information
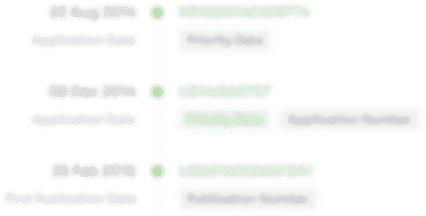
- R&D
- Intellectual Property
- Life Sciences
- Materials
- Tech Scout
- Unparalleled Data Quality
- Higher Quality Content
- 60% Fewer Hallucinations
Browse by: Latest US Patents, China's latest patents, Technical Efficacy Thesaurus, Application Domain, Technology Topic, Popular Technical Reports.
© 2025 PatSnap. All rights reserved.Legal|Privacy policy|Modern Slavery Act Transparency Statement|Sitemap|About US| Contact US: help@patsnap.com