Parameter optimization-based TE process fault diagnosis method for deep belief network model
A deep belief network and fault diagnosis technology, applied in instrumentation, electrical testing/monitoring, control/regulation systems, etc., can solve problems such as longer training time, reduced network generalization ability, and difficulty in convergence.
- Summary
- Abstract
- Description
- Claims
- Application Information
AI Technical Summary
Problems solved by technology
Method used
Image
Examples
Embodiment Construction
[0073] The specific implementation manner and working principle of the present invention will be further described in detail below in conjunction with the accompanying drawings.
[0074] from figure 1 It can be seen that a TE process fault diagnosis method based on a parameter-optimized deep belief network model follows the following steps:
[0075] Step 1: Take the TE process as the research object to carry out experimental simulation, obtain the simulation data, and divide the simulation data into training set data samples and test set data samples;
[0076] The specific content of the test simulation in step 1 is:
[0077] From all observed variables in the TE process, randomly select a gc observed variables as output variables, A-a gc An observed variable is used as a control variable;
[0078] In this embodiment, software environment: Matlab2016a, Windows7 operating system; hardware environment: CPU2.20GHz, memory 8GB, 750G hard disk.
[0079] In this embodiment, com...
PUM
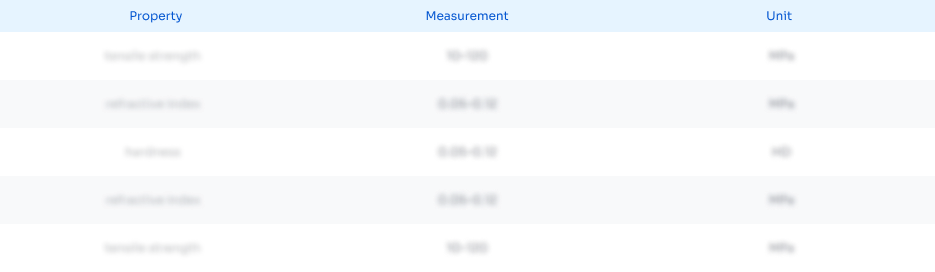
Abstract
Description
Claims
Application Information
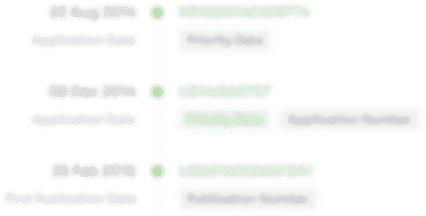
- R&D Engineer
- R&D Manager
- IP Professional
- Industry Leading Data Capabilities
- Powerful AI technology
- Patent DNA Extraction
Browse by: Latest US Patents, China's latest patents, Technical Efficacy Thesaurus, Application Domain, Technology Topic, Popular Technical Reports.
© 2024 PatSnap. All rights reserved.Legal|Privacy policy|Modern Slavery Act Transparency Statement|Sitemap|About US| Contact US: help@patsnap.com