Vehicle trajectory predicting method based on hybrid dynamic bayesian networks and gaussian process
A dynamic Bayesian and Gaussian process technology, applied in the direction of control devices, etc., can solve the problems of not considering vehicle driver information, poor interpretability, and ignoring influences.
- Summary
- Abstract
- Description
- Claims
- Application Information
AI Technical Summary
Problems solved by technology
Method used
Image
Examples
Embodiment Construction
[0101] The present invention will be further described below in conjunction with the accompanying drawings and embodiments.
[0102] Such as figure 1 As shown, the vehicle trajectory prediction method based on hybrid dynamic Bayesian network and Gaussian process includes the following steps:
[0103] Step 1. Build a natural driving database;
[0104] Establish a test set of surrounding vehicle-related sequence information, road-related sequence information and traffic-related sequence information collected by the automatic driving vehicle perception system, and a training set for calibrating driving intention and driving characteristics on the above information; wherein, the test set includes a mixture of The test set of dynamic Bayesian network and the test set of Gaussian process; The training set includes the training set of hybrid dynamic Bayesian network and the training set of Gaussian process;
[0105] The related sequence information of surrounding vehicles includes ...
PUM
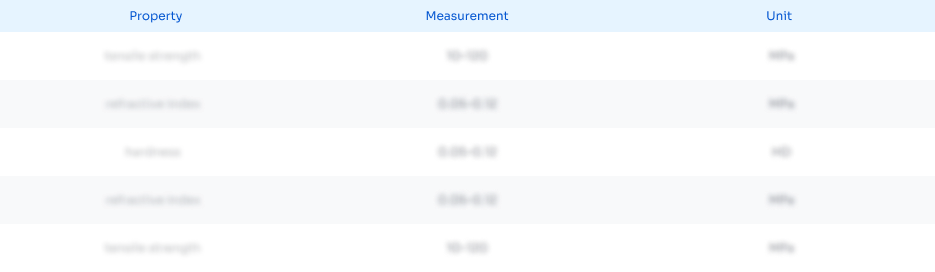
Abstract
Description
Claims
Application Information
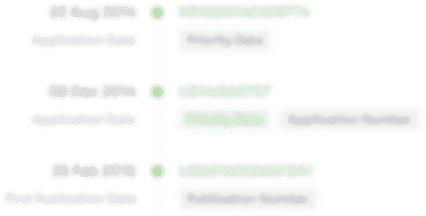
- R&D Engineer
- R&D Manager
- IP Professional
- Industry Leading Data Capabilities
- Powerful AI technology
- Patent DNA Extraction
Browse by: Latest US Patents, China's latest patents, Technical Efficacy Thesaurus, Application Domain, Technology Topic.
© 2024 PatSnap. All rights reserved.Legal|Privacy policy|Modern Slavery Act Transparency Statement|Sitemap