Multi-fault diagnosis modeling method of hybrid convolution neural network-driving lithium battery
A convolutional neural network and fault diagnosis technology, applied in biological neural network models, neural learning methods, neural architectures, etc., which can solve the problem of insufficient refined extraction of fault features, incomplete high-value fault data, and ineffective deep neural networks. At the same time, it can diagnose problems such as energy efficiency and improve reliability and safety.
- Summary
- Abstract
- Description
- Claims
- Application Information
AI Technical Summary
Problems solved by technology
Method used
Image
Examples
Embodiment Construction
[0026] Embodiments of the present invention are described below through specific examples, and those skilled in the art can easily understand other advantages and effects of the present invention from the content disclosed in this specification. The present invention can also be implemented or applied through other different specific embodiments, and the details in this specification can also be modified or changed based on different viewpoints and applications without departing from the spirit of the present invention. It should be noted that the illustrations provided in the following embodiments are only schematically illustrating the basic idea of the present invention, and the following embodiments and the features in the embodiments can be combined with each other under the condition of no conflict.
[0027] Wherein, the accompanying drawings are for illustrative purposes only, and represent only schematic diagrams, rather than physical drawings, and should not be const...
PUM
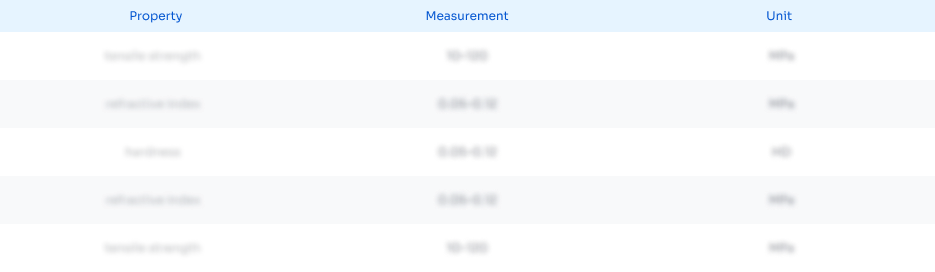
Abstract
Description
Claims
Application Information
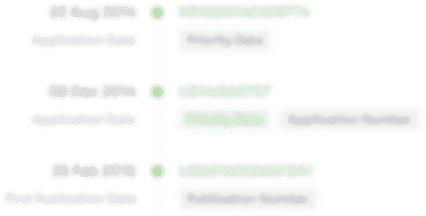
- R&D Engineer
- R&D Manager
- IP Professional
- Industry Leading Data Capabilities
- Powerful AI technology
- Patent DNA Extraction
Browse by: Latest US Patents, China's latest patents, Technical Efficacy Thesaurus, Application Domain, Technology Topic, Popular Technical Reports.
© 2024 PatSnap. All rights reserved.Legal|Privacy policy|Modern Slavery Act Transparency Statement|Sitemap|About US| Contact US: help@patsnap.com