Pecan common pest recognition method based on deep learning
A deep learning, pecan technology, applied in character and pattern recognition, instruments, computer parts, etc., can solve problems such as insufficient data sample size, large fluctuations in model fit, low recognition accuracy, etc., to achieve automatic Recognition and classification, improving recognition accuracy, and the effect of high recognition accuracy
- Summary
- Abstract
- Description
- Claims
- Application Information
AI Technical Summary
Problems solved by technology
Method used
Image
Examples
Embodiment 1
[0067] A kind of method for identifying and classifying hickory pests based on deep learning of the present embodiment, refer to figure 2 , including the following steps:
[0068] Collect image data of hickory pest samples;
[0069] The hickory pest sample image data is processed to obtain the processed hickory pest sample image data set;
[0070] Use the VGG convolutional neural network model and optimize the VGG convolutional neural network model:
[0071] VGG convolutional neural network model reference figure 1 , mainly composed of input layer, convolutional layer, pooling layer, fully connected layer, and Softmax layer. VGGNet can be divided into 5 layer groups, including Conv1 convolutional layer group 1, Conv2 convolutional layer group 2, Conv3 convolutional layer group 3, Conv4 convolutional layer group 4 and Conv5 convolutional layer group 5, fully connected layer including FC6 Fully connected layer 6 and FC7 fully connected layer 7. Each layer group contains 1 ...
Embodiment 2
[0078] A kind of hickory pest identification and classification method based on deep learning of the present embodiment, based on embodiment one, collects the image data of different growth stages of hickory pest, for example collects the image data of pest from egg to larva to adult stage, is used for training The model avoids the error in model detection caused by the large difference in the body characteristics of pests in different growth stages. Of course, the types of pests are different and the growth stages of pests are different. In addition, the color of the hickory pest sample image is reserved according to the situation, because the pests not only have differences in shape and texture, but color is also an important factor affecting the recognition accuracy. Therefore, in the process of data processing, the input image data is not converted to grayscale. image, but retains the values of the RGB three color channels of the image.
Embodiment 3
[0080] A kind of hickory pest identification classification method based on deep learning of the present embodiment, based on embodiment 2, hickory pest sample image data is processed, obtains the hickory pest sample image data set after processing, comprises the following steps:
[0081] Dealing with erroneous and duplicate data in hickory pest sample image data;
[0082] Using data augmentation to expand the image data of hickory pest samples.
[0083]Use data enhancement to expand the image data of hickory pest samples: After screening, the number of samples of each insect is different. In order to improve the classification accuracy of the network, make the performance of the network better, and prevent problems such as overfitting, this implementation For example, data enhancement is used to expand the amount of image data of the above-mentioned hickory pest sample. By operating the image data of the above-mentioned hickory pest sample, for example, in this embodiment, th...
PUM
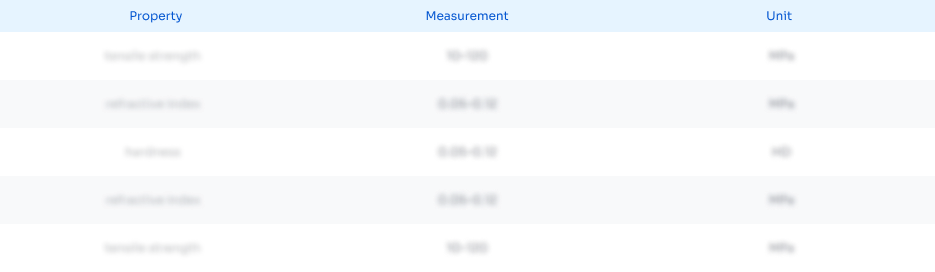
Abstract
Description
Claims
Application Information
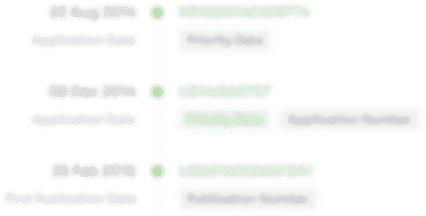
- R&D Engineer
- R&D Manager
- IP Professional
- Industry Leading Data Capabilities
- Powerful AI technology
- Patent DNA Extraction
Browse by: Latest US Patents, China's latest patents, Technical Efficacy Thesaurus, Application Domain, Technology Topic, Popular Technical Reports.
© 2024 PatSnap. All rights reserved.Legal|Privacy policy|Modern Slavery Act Transparency Statement|Sitemap|About US| Contact US: help@patsnap.com