Deep learning model reasoning acceleration method based on cooperation of edge server and mobile terminal equipment
An edge server and deep learning technology, applied in neural learning methods, biological neural network models, physical implementation, etc., can solve problems such as delay and energy consumption, huge computing and storage overhead, and mobile devices cannot provide performance. To achieve the effect of shortening the reasoning delay
- Summary
- Abstract
- Description
- Claims
- Application Information
AI Technical Summary
Problems solved by technology
Method used
Image
Examples
Embodiment
[0046] This embodiment discloses a deep learning model inference acceleration method based on the collaboration of an edge server and a mobile device. The method implements accelerated deep learning model inference by combining model segmentation and model simplification. The following will introduce model segmentation and model simplification, and finally show the execution steps of the deep learning model inference acceleration method in actual operation.
[0047] (1) Model segmentation
[0048] For the current common deep learning model, such as convolutional neural network, it is formed by superimposing multiple layers of neural network layers, including convolutional layer, pooling layer, fully connected layer, etc. It is very difficult to directly run a neural network model on a resource-constrained terminal device due to the need to consume a large amount of computing resources, but because the computing resource requirements of different neural network layers and the s...
PUM
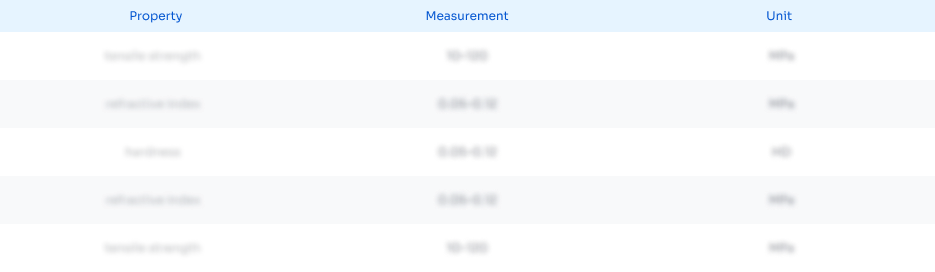
Abstract
Description
Claims
Application Information
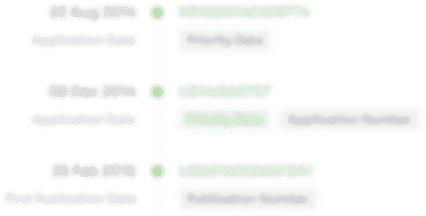
- R&D Engineer
- R&D Manager
- IP Professional
- Industry Leading Data Capabilities
- Powerful AI technology
- Patent DNA Extraction
Browse by: Latest US Patents, China's latest patents, Technical Efficacy Thesaurus, Application Domain, Technology Topic, Popular Technical Reports.
© 2024 PatSnap. All rights reserved.Legal|Privacy policy|Modern Slavery Act Transparency Statement|Sitemap|About US| Contact US: help@patsnap.com