Airborne laser point cloud classification method based on local and global depth feature embedding
A deep feature and airborne laser technology, applied in the field of point cloud classification, can solve problems such as ignoring context constraints and only considering spatial regularity, so as to optimize marking, refine classification results, and improve robustness
- Summary
- Abstract
- Description
- Claims
- Application Information
AI Technical Summary
Problems solved by technology
Method used
Image
Examples
Embodiment
[0050] The proposed point cloud classification method proposed by the present invention includes three main steps, as figure 1 Shown: (1) multi-scale deep feature learning; (2) feature embeddings that preserve local space and feature domain; (3) and graph-based global optimization.
[0051] (1) In the first step, the whole city scene is divided into fragments with different sizes, and these fragments are down-sampled to a fixed number to meet the input requirements of the designed network (such as figure 2 shown). These fragment samples of different scales are then fed into PointNet++ to generate initial classification probabilities, as well as deep features for each point, which include not only point-based features but also regional features for each chip. The scene point cloud segmentation here uses a segmentation method based on a combination of supervoxels and region growth.
[0052] (2) In the second step, to further improve the clarity and spatial correlation of extr...
PUM
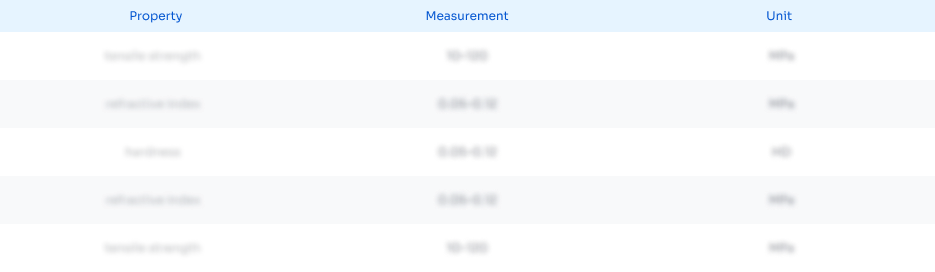
Abstract
Description
Claims
Application Information
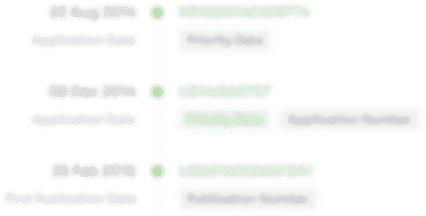
- R&D Engineer
- R&D Manager
- IP Professional
- Industry Leading Data Capabilities
- Powerful AI technology
- Patent DNA Extraction
Browse by: Latest US Patents, China's latest patents, Technical Efficacy Thesaurus, Application Domain, Technology Topic.
© 2024 PatSnap. All rights reserved.Legal|Privacy policy|Modern Slavery Act Transparency Statement|Sitemap