Medical dataset characteristic dimension reduction method based on subspace learning
A subspace learning and medical data technology, applied in the field of big data technology and machine learning, can solve the problems of not considering the importance of global discriminant information, the optimal solution cannot be solved by eigenvalue decomposition, and the eigenvector is inaccurate, etc., to achieve the goal of choosing The method is simple and achievable, convenient for feature selection, and has good robustness
- Summary
- Abstract
- Description
- Claims
- Application Information
AI Technical Summary
Problems solved by technology
Method used
Image
Examples
Embodiment
[0099] The present invention is based on the medical data set feature dimensionality reduction method of subspace learning, comprises the following contents:
[0100] 1. Construct the original high-dimensional data matrix X and the label column according to the medical data set to be analyzed.
[0101] The data set used in this embodiment is the ARCENE data set, which comes from human serum mass spectrometry. The sample size of the ARCENE dataset is 900, and the feature dimension is up to 10000. The task is a binary classification problem that aims to distinguish people with cancer (labeled +1) from normal people (labeled -1). The entire dataset is merged from two prostate cancer datasets and one ovarian cancer dataset from the National Cancer Institute (NCI) and Eastern Virginia Medical School (EVMS). The data has no missing values and approximately 44% of the samples are positive. The dataset consists of three parts: a training dataset with 100 samples, a validation dat...
PUM
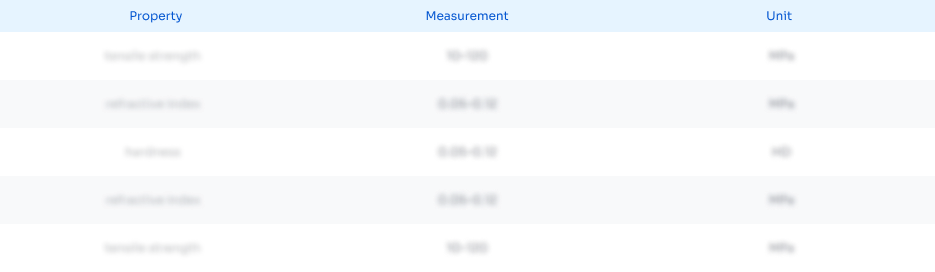
Abstract
Description
Claims
Application Information
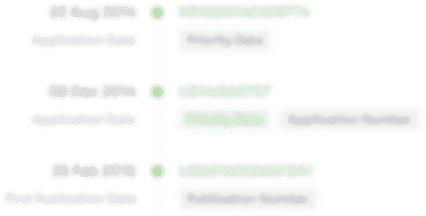
- R&D Engineer
- R&D Manager
- IP Professional
- Industry Leading Data Capabilities
- Powerful AI technology
- Patent DNA Extraction
Browse by: Latest US Patents, China's latest patents, Technical Efficacy Thesaurus, Application Domain, Technology Topic, Popular Technical Reports.
© 2024 PatSnap. All rights reserved.Legal|Privacy policy|Modern Slavery Act Transparency Statement|Sitemap|About US| Contact US: help@patsnap.com