Photovoltaic module fault diagnosis method, system and device based on deep convolutional adversarial network
A technology of deep convolution and photovoltaic modules, applied to biological neural network models, computer components, instruments, etc., can solve problems such as time-consuming and labor-intensive, low resolution of fault data samples, and difficulties in accurate mathematical models
- Summary
- Abstract
- Description
- Claims
- Application Information
AI Technical Summary
Problems solved by technology
Method used
Image
Examples
Embodiment Construction
[0063] The application of this method will be described in detail below with reference to the accompanying drawings.
[0064] The present invention solves the existing technical problems through the following technical solutions:
[0065] Such as figure 1 As shown, the photovoltaic module fault diagnosis method based on deep convolutional confrontation network includes the following steps:
[0066] Step 1, collect the image information collected by the photovoltaic module, filter out the fault data representing the fault type, mark it as the original sample, and set part of the fault data in the original sample as the training sample x;
[0067] Step 2, in TensorFlow (a kind of software based on data flow programming), construct deep convolution confrontation network model, described depth convolution confrontation network model comprises generator G and discriminator D;
[0068] Input a 100-dimensional noise vector z to the generator G, change the 100-dimensional noise vect...
PUM
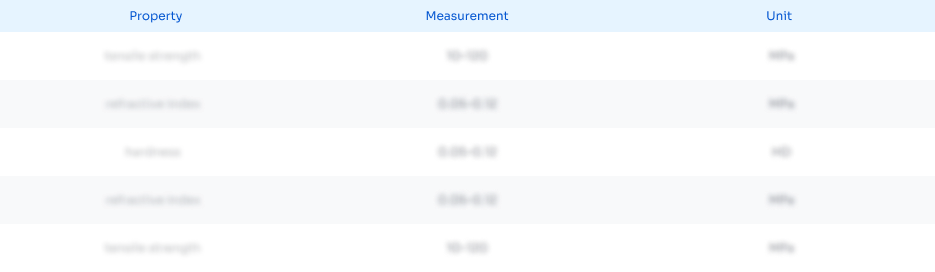
Abstract
Description
Claims
Application Information
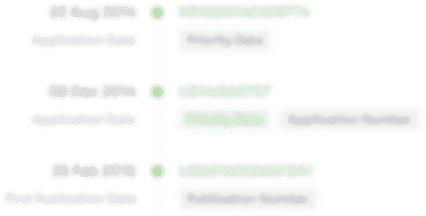
- R&D
- Intellectual Property
- Life Sciences
- Materials
- Tech Scout
- Unparalleled Data Quality
- Higher Quality Content
- 60% Fewer Hallucinations
Browse by: Latest US Patents, China's latest patents, Technical Efficacy Thesaurus, Application Domain, Technology Topic, Popular Technical Reports.
© 2025 PatSnap. All rights reserved.Legal|Privacy policy|Modern Slavery Act Transparency Statement|Sitemap|About US| Contact US: help@patsnap.com