LSTM-based automatic multi-classification identification method for ballistocardiogram signals
A technology of cardiac shock signal and identification method, applied in the field of deep learning physiological signal classification, can solve the problem of relying on manual design and low classification accuracy, and achieve the effect of reducing manual feature extraction work and reducing the interference of accuracy.
- Summary
- Abstract
- Description
- Claims
- Application Information
AI Technical Summary
Problems solved by technology
Method used
Image
Examples
Embodiment
[0067] Such as figure 1 As shown, an LSTM-based automatic multi-classification recognition method for cardiac shock signals includes the following steps:
[0068] S1. Acquiring data set training samples;
[0069] S2. Using the wavelet threshold denoising method to preprocess the data set training samples obtained in step S1 to obtain pure cardiac shock signals;
[0070] S3. The cardiac shock signal obtained in step S2 is matched with the adaptive threshold to complete the positioning of the IJK wave, and the cardiac beat interception of the cardiac shock signal is obtained;
[0071] S4, build the LSTM network model, the heartbeat interception of the cardiac shock signal obtained in step S3 is used as the input data of the LSTM model, and the network model is trained and tested;
[0072] S5. During the training process, the backpropagation algorithm is used to optimize the weight of the constructed LSTM network model, so that the network converges to the global optimum;
[0...
PUM
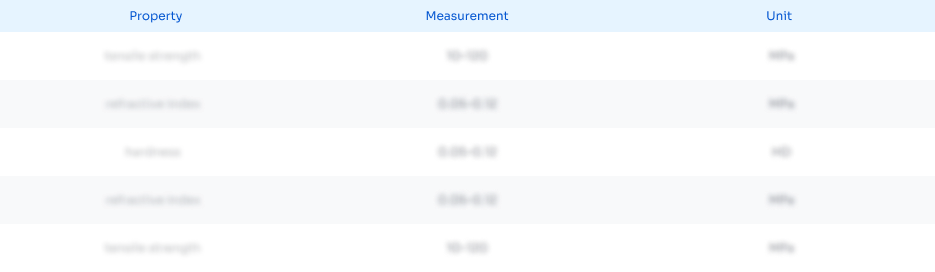
Abstract
Description
Claims
Application Information
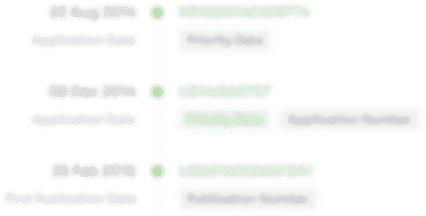
- R&D Engineer
- R&D Manager
- IP Professional
- Industry Leading Data Capabilities
- Powerful AI technology
- Patent DNA Extraction
Browse by: Latest US Patents, China's latest patents, Technical Efficacy Thesaurus, Application Domain, Technology Topic, Popular Technical Reports.
© 2024 PatSnap. All rights reserved.Legal|Privacy policy|Modern Slavery Act Transparency Statement|Sitemap|About US| Contact US: help@patsnap.com